Where AI and big data can help to analyse the risks
Advanced analytical techniques and increased data-handling capacity are extending the use of more complex models to new areas in many industries, in particular financial services. This has heightened regulatory concern about excessive reliance on models. For they have their limits. Many, by design, work well only under stable conditions. When faced with structural changes or sudden disruptions, their reliability can be significantly compromised. Indeed, during the COVID-19 pandemic many models have broken down. Against this background, financial service companies could benefit more than ever from developing forward-looking, scenario-based analyses and integrating them into their decision-making processes. By doing so, companies will be better informed and thus better positioned to handle both business as usual and times of stress. Big data and AI can help in this task by identifying and using real-time information on client’s behaviours, which can benefit decision making and response plans.
The attraction of models
Financial institutions rely heavily on statistical and mathematical models. Indeed, their use has grown dramatically in the past few years, thanks in part to the spread of digitalisation and automation. These models are embedded in a host of processes serving a wide range of purposes. They help banks meet regulatory requirements by estimating capital requirements, provisioning needs under IFRS 9 and stress-testing. They also help with myriad aspects of running the business, including budgeting, planning and risk assessment and management.
Meanwhile, innovative analytical methods have paved the way for financial institutions to introduce increasingly sophisticated models to raise their game in areas such as fraud detection. For example, we are all familiar with the design of traffic lights around the world (CAPTCHA) to distinguish people from bots. A combination of big data and artificial intelligence can show at once whether the response is coming from a person or – fraud alert – from a machine.
The popularity of models, built on vast amounts of structured and unstructured data, has risen so sharply that a new discipline has sprung up in recent years to mitigate the risks stemming from their use: Model Risk Management (MRM).
Regulators in particular have been concerned about heavy reliance on models. The Federal Reserve took the lead in 2011 with a regulation requiring financial companies to ensure comprehensive oversight of the models they use. The rule provided an early definition of what should be understood as a model, along with guidance on how to manage the risks their use entails. An important element within this management framework is a requirement for decision makers to understand the limitations of the models. To take an example, a model of projected mortgage defaults in Spain will probably not be applicable in Britain or Germany, at least not with the same level of confidence.
One limitation of many models, including those based on advanced techniques such as big data or artificial intelligence, is that they are designed to work well in a stable environment. Many, by construction, predict the future based on the past. It’s like driving by staring through the rear-view mirror: when the road is straight, what you see is a relatively good approximation of the future. So, a typical model, by looking at relationships based on existing data, necessarily projects a stable future. But, to continue the driving analogy, when a bend in the road is coming up, steering via the mirror is useless. It’s positively dangerous. And we now have a long, winding road ahead of us with plenty of bends and curves.
Against this background, managers have realised that models cannot be trusted in times of crisis to make decisions about many aspects of a bank’s operations or some requirements like provisioning. Thus banks - but not only banks - could benefit from adjusting the data and methodologies they use to adapt to the changed circumstances.
Many models are too rigid to incorporate high-frequency data that would allow them to be recalibrated. Even if that were possible, there would be no guarantee of success because the models would still be using past information to approximate the future.
There are several examples of widely used models that have broken down in the current environment:
- Loan application credit scorings – COVID-19 has weakened the value of the data used in credit rating systems. For example, rating an SME based on its balance sheet and income statements (produced with a significant delay), or even with more up-to-date information, fails to provide an accurate assessment of its current situation and chances of survival. Notably, there are non-financial elements that models tend not to capture. For example, does the current crisis change not only a customer’s capacity to repay but also their willingness to repay?
- Risk estimation models – The same applies to risk parameters such as probabilities of default (PD) or loss given default (LGD), parameters used for provisioning and capital requirements.
- Financial planning models – Most financial planning models are based on traditional segmentation methods. Projections of net interest income are modelled on the basis of products (mortgages, credit cards, sight deposits, etc) and ignore crucial factors such as the sector in which the customer works and their capacity to keep earning by working from home.
Another drawback of these models is that they take no account of the various relief programmes that governments have launched to cushion the economic impact of the pandemic on companies and employees.
What to do?
Navigating through crises like COVID-19 is particularly challenging because of the radical uncertainty associated with the pandemic. The repercussions of protracted lockdowns, social distancing and unprecedented economic disruption involve complex dynamics and chain reactions. Traditional backward-looking risk assessments cannot anticipate accurately the full fallout of the disease. To do that, it is necessary more than ever to develop forward-looking, scenario-based analyses. Going back to our driving analogy, we need to start peering through the windscreen while glancing back now and then in the mirror.
Behavioural elements can play a significant role in such analyses. For example, some borrowers are responsible while other are may be more willing to build up debt at the moment. Is the crisis likely to widen the gulf between the two categories?
An adapted approach is needed in regard to stress-testing techniques used by financial institutions to help determine the economic impact of the coronavirus. Stress-testing typically involves coming up with a central GDP scenario and, extrapolating from past experiences, using it to anticipate gains and losses on the loan book and asset portfolio. But such a traditional model risks generating more problems than solutions because this crisis is different. A fresh way of looking at the economic shock of COVID is called for.
A downturn is normally triggered by a shock in one or more specific sectors of the economy. In a typical recession, the immediate direct impact may be relatively small (e.g. job losses in banking during the Global Financial Crisis) before the after-effects ripple through the rest of the economy – sometimes in ever-bigger waves. The COVID crisis is a different beast altogether. The direct effects of the economic lockdown are massive and widespread. Traditional models may well fail to capture them.
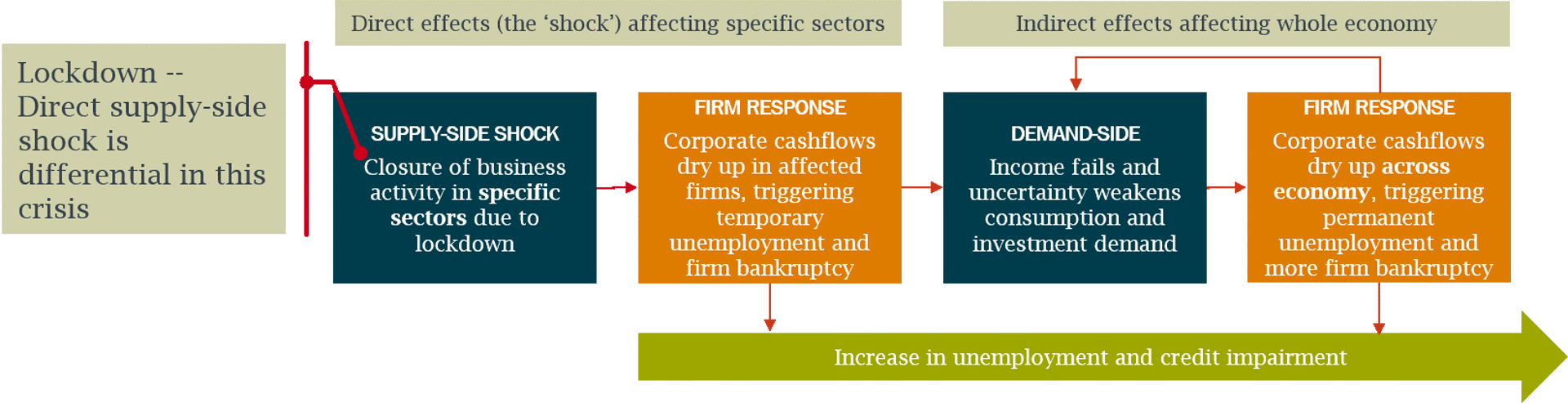
Instead, banks could benefit from generating a bottom-up analysis of their different customer segments based not on the products they have taken out – the conventional approach – but how they will be affected by the economic shock.
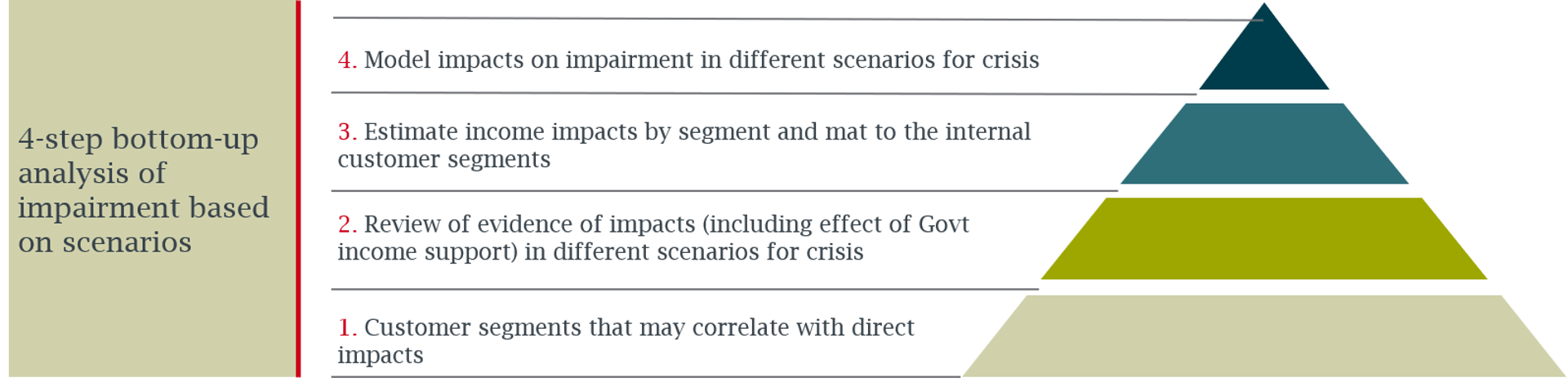
Such an analysis would be carried out in the following sequence:
- Identify the customer – Establish from the bank’s client base those customers most likely to be affected by the direct impact of the lockdown. The determining factors are likely to be occupation, employment status, income, region, age and payment behaviour.
- Review the direct shock under different scenarios – These would incorporate forecasts for the economy, as well as specific sectors and regions, mapped against the segmentation of the bank’s customers. The scenarios should also reflect the impact of government income support policies.
- Match the estimated income impact on affected groups to the customer segments within the bank’s database - Using the appropriate mapping, the impact could be generated under different scenarios of the extent and duration of the lockdown, say three and six months.
- Model impairment in various scenarios – Once the direct effects have been generated, existing models can be used to translate the anticipated income shock into impairment effects for each segment and the bank’s book overall.
Big data and AI could help in this task. Big data has allowed for the storage, management and analysis of information on clients’ behaviours in real-time. In a crisis situation, this can give companies more items to monitor in order to identify weaknesses and prove useful in many ways including the identification of shifting consumption patterns and trends, the elaboration of real-time alerts and the generation of behavioural segmentations. Together with advanced analytical technics, it can inform decision making and targeting of products and services and deliver a quick and accurate response plan.
Conclusion
The COVID-19 pandemic has exposed the shortcomings of the models on which banks have come to rely so heavily. The models need to be supplemented with forward-looking, scenario-based analytical techniques underpinned by big data and artificial intelligence. A bank with access to the full suite of client data, harnessed to the ability to break it down into different segments for analysis, will be in a strong position to make well-informed decisions, especially in a time of crisis.
Investigating and acquiring the required technology takes time, effort and money. But banks should think of the investment as necessary insurance, not as a luxury. And, unlike insurance, advanced analytics will pay off not just during a crisis but also when it’s business as usual, by allowing managers to segment the bank’s customer base ever more finely and to tailor their products and services accordingly.