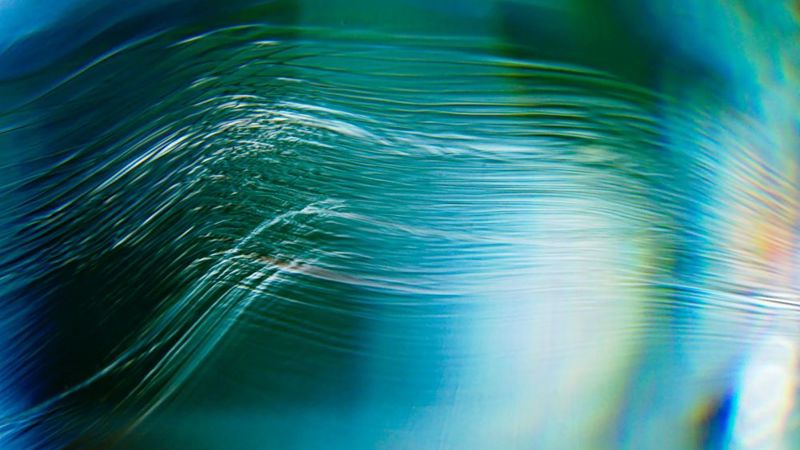
Opportunities to drive efficiency and innovation
For many companies, in the water sector and beyond, collecting and analysing historical performance data in order to unlock operational insights has long been a driver of efficiency and innovation.
In the past, data collection and analysis were time-consuming, labour-intensive and often unfruitful. But now, AI and machine learning create new and exciting opportunities.
Imagine being able to predict the next mains burst.
For many companies, in the water sector and beyond, collecting and analysing historical performance data in order to unlock operational insights has long been a driver of efficiency and innovation. However, to date, the potential benefits of this approach have not been fully realised. Vast amounts of data, covering everything from the real-time condition of underground assets to customer sentiment on social media posts, tend to go largely unrecorded and unanalysed. The data that is collected may be inaccurate, incomplete or simply not fit for purpose, and the techniques used to analyse it may not prove to be particularly insightful. In the past, data collection and analysis were time-consuming, labour-intensive and often unfruitful, but now, technological progress creates new, exciting opportunities. Automated data collection, such as through the use of sensors and AI, and the ability to analyse enormous volumes of data through machine learning, represent a step change in this approach to unlocking insights to drive efficiency.

This presents an opportunity for companies to put an even greater focus on data analytics to improve service quality and cost efficiency – although it will require more time and cultural change from the sector. And, in turn, Ofwat will need to reflect on how best to incorporate this potential into its approach to regulation in order to promote better market outcomes for consumers.
In this bulletin we provide the following high-level thoughts:
- We describe a vision of the water company of the future; and
- We consider the steps and actions that can be made today – by companies and Ofwat – that will determine how quickly we get there.
The water company of the future
We envisage a future where each water company develops its own ‘digital twin’, i.e. a virtual data-driven replica of the business supplemented with enormous amounts of granular data from multiple sources to replicate the environment in which it operates. A digital twin will provide a unique opportunity for companies to analyse statistical relationships with great accuracy and precision. Encouragingly, some companies are already developing their own twins.

To develop a digital twin, companies will need to invest in various sensor-like technologies to map and take readings of their infrastructure, and supplement this with data pulled in from other sources. This will always be a moving target, and as more and more data becomes available, the twin will need to be continually updated.
Having developed a digital twin, the techniques to analyse this data will also grow more and more sophisticated, beyond just charts and tables in spreadsheets to more complex machine learning algorithms.
For example, companies may wish to find the optimal maintenance schedule that reduces the cost of running an asset. Rather than formulating simple hypotheses and testing them one by one, an algorithm could be given free rein to test millions of multi-variable models with non-linear relationships, without being explicitly programmed to do so. By allowing complex combinations of these variables in what is known as a “non-parametric model”, relationships may be found that the company did not even think to consider. Similar analysis could be targeted at other objectives, such as improving service quality and cost efficiency.
Such techniques can be applied to datasets that would otherwise overwhelm a human analyst manually looking for relationships. However, the algorithms cannot be left to their own devices: expert input will still be critical to build and interpret such models. At a minimum, the model will need to be given an objective. For example, which measures of service quality are most important? Getting the most relevant results from a model will also require the analyst to feed their own knowledge into the model. For example, by itself, a machine has no concept of causality: it may identify that actions made in the future drive outcomes in the past. The model will certainly not “know” the physical or economic relationships that may be clear to an analyst. However, with the right techniques, the algorithm can be guided to produce much more accurate predictions than the analyst alone could make.

Making this future a reality
The future may not look exactly like this, but we do believe it is the direction of travel. Indeed, aspects of this future already exist today. Some companies already have sophisticated rostering software and investment optimisation models that carry out some of the analysis described above. These are useful stepping stones to bring us closer to the vision of a digital twin, and they highlight how companies are already making technological and cultural changes to embrace automation and technology-led optimisation. But they tend to be only as good as the data that is used to populate them. The vision set out above may be some years away and will require substantial investment, but it illustrates how data insights have the potential to transform the way we plan, deliver and analyse water services.
Companies are already moving towards this future. However, investment in the technologies mentioned earlier will come at significant cost and effort over many years and multiple regulatory periods. And the benefits of these investments will also be realised over many regulatory periods. The PR19 final determinations have set stretching cost and service quality targets for the sector, and the issues raised by the COVID-19 pandemic mean that investment in data – which in the short run is not essential to operations and largely speculative in nature for now – will be difficult to justify. The current regulatory approach creates short-term incentives that may not be compatible with the multi-period costs and benefits described above.
But, in our view, more and better data is essential to keep driving long-term efficiency. Companies should be encouraged to invest now. From a regulatory perspective, to facilitate moving towards this future, we believe that the key questions for the water sector are:
- Can companies be confident that the future regulatory approach makes efficient investment in this area worthwhile and reflects the long-term benefits of the actions?
- How can the innovation fund play a role to stimulate investment?
- How can existing regulatory incentives be modified?
- Can the methodology for PR24 be more explicit in rewarding companies for efforts in this area?
In parallel, companies should continue to consider whether there are any easy wins that could be already implemented today, especially as certain investments could already help them outperform relative to the stretching targets set at PR19. Specifically:
- Could routine investments be upgraded to include smarter technologies, such as sensors? Some of the cost could potentially be shared with other utility network operators.
- Could companies do more to combine and operationalise the datasets they already have and also reach out to third parties to combine datasets from external sources?
- Could companies experiment more with machine-learning specialists?
- Could companies share among themselves best practice not only in the water industry but also lessons learned from other sectors?
While this vision may take time to materialise, it is the decisions that are made today, by companies and by Ofwat, that will determine how quickly we get there.