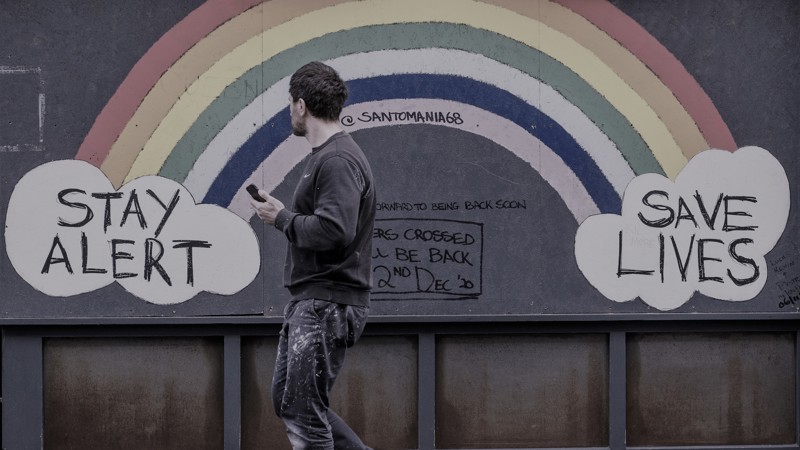
The coronavirus crisis has temporarily changed the policy development cycle. Near-time information on disease prevalence and population mobility has shaped public health policy. Weekly estimates of unemployment claims have helped track the effectiveness of the furlough scheme.
Near-time data has enabled governments to monitor activity and introduce or tweak policies accordingly. It has also allowed for estimates of early impact - crucial when understanding policy effectiveness is a matter of life and death.
Policymakers should not let these lessons lapse. They should continue to use near-time information to inform recovery efforts and wider policymaking priorities such as levelling up.
Near-time data can improve decision-making and drastically cut the time from policy formulation to policy assessment
Near-time data offers close to real-time insights into economic and social trends. VAT receipts, traffic, footfall and credit card spending are examples of near-time data pipelines developed by government and commercial stakeholders. Near-time evidence contributes to better policy trade-offs and helps measure outcomes more quickly. We estimate that, for business support policies, going from policy formulation to assessment could be up to 3x shorter with near-time data.
Figure 1: Example of potential time benefits from near-time data on business support policy evaluation cycle

Policy cycles comprise six components (ROAMEF) and are the official way the UK Government develops, implements and evaluates policies, as part of its Green Book guidance. Take the policy cycle for a business support intervention without near-time data as an example:
Rationale - A case is made that Government should intervene to support small businesses. The case is based on evidence from business microdata, economic analysis of market failures and views from constituents and civil society. The time taken to conduct business surveys means at least a year is needed to flesh out an evidence-based rationale for intervention.
Objectives - Objectives are set against which the policy is appraised and evaluated, for example reducing the number of business closures due to a lack of access to finance.
Appraisal - Different support options are appraised according to their costs and likely impacts, with a focus on the associated risks and assumptions. For example, block grants to cash-constrained firms are compared with outreach programmes to make small businesses aware of their financing options and ways to improve cashflow.
Monitoring - Once an option is chosen, a logic model is developed to understand pathways from policy inputs to the ultimate impacts they have. Measures are implemented to support small businesses. They are monitored regularly to ensure they are carried out according to plan and the relevant stakeholders are benefiting.
Evaluation - Several years after the short-, medium- and long-term impacts are felt, business census data is released and the value for money of the policy is evaluated.
Feedback – Once the evaluation is complete, policymakers understand what has worked and what hasn’t. If the objectives have not been reached, the policy cycle commences again to assess whether further policy action is needed.
As shown in Figure 2, near-time data can significantly enhance four aspects of the policy cycle:
- Setting the policy rationale – Near-time data can improve policy targeting and overall effectiveness. It can help answer questions such as: Which are the firms most in need of support? Where are they located? What levers do they have in common that policymakers can pull? Near-time information will speed up the identification of areas for intervention, bringing forward the policy appraisal stage.
- Appraisal - The costs of policy options are best assessed with up-to-date inputs – not with business and consumer surveys from previous years. When thinking about a business support programme, near-time data is key to establishing how many firms currently need help and are likely to take up an offer of aid. This will generate a more precise cost estimate, which in turn will enhance appraisal of the programme.
- Monitoring – Near-time monitoring lets policymakers quickly learn lessons from the execution of a policy and to make corresponding course corrections. Are businesses taking up the support as intended? Are certain sectors or areas doing so more quickly than others? Are there unintended consequences?
- Evaluation – Business census data takes years to be published. Near-time information on changes in footfall, business insolvency data and tax/VAT receipts could speed up the assessment of the short- and medium-term impacts of a policy intervention by several years.
Figure 2: Near-time data benefits across the policy cycle

Taken together, near-time data shortens the time from rationale to feedback by several years. End-of-project evaluations as still necessary to quantify long-term impacts in the round and measure the value for money of a policy. However, speeding up the policy cycle will help inform the likely success of the policy.
Measuring early impacts is key and near-time data helps with this. If short-term effects are being seen, there are grounds to expect long-term impacts too. Likewise, if the policy makes no short-term difference, there is scope to correct course early in the evaluation. Without near-time data, such policy adjustments are not possible.
Key lessons have been learned...
Two features make near-time data useful.
First, open and easily accessible data has allowed government and private stakeholders to monitor key outcomes quickly without needing to request access to administrative data or wait for conventional surveys. Multiple data sources, such as various mobility indexes from government and the private sector, were compared to provide robust evidence.
Second, thinking about key proxies to inform outcomes has been critical. This has involved setting out the ways in which a policy makes an impact. For example, indexed transport usage approximates the degree of population mixing, which is an important goal of non-pharmaceutical interventions to reduce virus transmission. As for company support schemes, information on monthly voluntary dissolution applications, real-time tax information and weekly job adverts proxy business survival.
To be effective, near-time data needs to be considered at the outset of policy-making to embed it as deeply as possible in the policy cycle.
… which can be applied to a wide range of policy areas
Government is embarking on significant initiatives, such as aiming for net zero carbon emissions, increasing R&D investment, levelling up and reforming social care, as well as striving to ‘build back better’ post-COVID. Incorporating near-time data in the policy cycle will lead to significant benefits for citizens and increase overall welfare.
There are two criteria for determining where policymakers can use near-time data to achieve the greatest impact.
First, the availability and usefulness of near-time data. The collection of near-time data requires significant infrastructure. However, some indicators have already been developed that can provide useful information, including those from the ONS Data Science Campus and private-sector datasets on retail footfall and population mobility.
Not all near-time indicators measure the exact outcome of interest - for example, information on near-time internet traffic developed by the ONS using data from internet exchange networks across the country. While very few policies will target internet usage as an outcome, this near-time measure is correlated with road traffic and commuting patterns. Specifically, the size of the reduction in traffic observed after 4pm proxies the extent to which commuters are travelling to and from their offices.
Understanding the proportion of people working from home in different parts of the country post-COVID will be important. Internet traffic data in near-time can shed light on the degree to which commuting has reverted to pre-pandemic levels.
Second, the length of the policy evaluation cycle. Near-time data shortens the cycle, which is especially important for policy interventions whose effects are felt in both the short and the long run. Normally, evaluation occurs towards the end of the cycle once the long-term impacts have materialised. For a multi-year policy this is several years after it is initially developed. Using near-time data helps measure the early effects more quickly, paving the way for timely tweaks to improve the ultimate outcomes of the policy.
In Figure 3, we map a subset of key current government policies on our criteria:
Figure 3: Near-time data policy matrix

Policies in the upper right quadrant are big wins. There is near-time data available and significant value in early-impact measurement. For multi-year policies, reducing the time taken to estimate early effects is crucial given their size. Considering the use of near-time data when setting out these policies will be an easy but important step. This will require putting forward a theory of change which explicitly ties outcomes to near-time datasets. It will also involve crafting evaluation methods which can interact with near-time data to estimate the causal effect of the policy.
Policies currently in the bottom right are areas for development. These are multi-year programmes which are likely to benefit from near-time data. However, such data on key outcomes has yet to be developed. Liaising with industry and governmental stakeholders to collect near-time data for these policies should be considered.
We explore policies in each of the four quadrants in turn, highlighting the contribution of near-time data to each.
It is worth noting that the matrix in Figure 3 is the view today. But there may be scope for policies to shift to other quadrants. Digital transformations of government functions and processes as well as increasingly open data may lead to new sources of near-time data. The precise outputs or outcomes of interest may lead to shorter or longer evaluation cycles. The matrix helps to illustrate options for combining alternative approaches to evaluation with alternative data sources – and where those might currently combine to allow real-time evaluation.
COVID-19 recovery – big win
The importance of extending near-time data already developed in response to the pandemic to aid the recovery is self-evident. Key indicators on retail footfall, company voluntary dissolutions, tax returns and mobility are already being collected and used. Long-term programmes such as the Cultural Recovery Fund would benefit from appraising policy options using near-time evidence and linking their outcomes to these data feeds to evaluate what impact they are having.
Active labour market policies to prevent long-term unemployment would benefit from near-time data on job postings as well as information on universal credit claimants. This is especially important in understanding short-term outcomes from the scaling back of the furlough scheme and changes to work.
To get people back into work after the pandemic, the government needs a firm grasp of the re-employment process in both the short and the long term. Near-time data can be very effective in helping policymakers understand what works in the short run.
Future of skills and lifelong learning – area for development
Policies such as life-long learning and reskilling are likely to become more relevant in future. Investing now in the capacity to evaluate such policies quickly will be repaid many times over. The effects of upskilling take several years to materialise, so harnessing near-time data to make prompt policy assessments can be particularly useful.
Several near-time datasets exist to identify which skills are in demand, such as those derived from scraping job-posting websites. However, less is known about the take-up of lifelong learning opportunities. It is therefore important to develop near-time data metrics to help provide answers so the effectiveness of the policies concerned can be evaluated swiftly. This could involve collaboration with education providers in the private and public sectors to understand the take-up of upskilling and reskilling courses and the destinations of course graduates.
Policies to boost export competitiveness following UK industry GHG reductions
Near-time data can be used to track changes in the export competitiveness of industry as a result of emission reduction policies. Data on shipping and VAT receipts could highlight the unfolding impact on exporters. A scoping study on the correlation between administrative exporter-level data and these near-time indicators would be an important first step. This would narrow the list of near-time datasets that best reflect the policy outcomes of interest.
Unlike levelling up, any impact on exporter competitiveness is a consequence of policy actions. Therefore, policy development is mitigatory and its evaluation cycle is shorter. Near-time data still has significant value by allowing more accurate appraisal and identifying quickly what works. However, the scope of the policy is necessarily smaller than vast longer-term initiatives such as levelling up and aiming for net zero.
Supporting incomes in retirement
Supporting income in retirement, though important, is unlikely to benefit as much from near-time data as other policies. This is because the relevant data pipelines have not been developed and policy is currently focused on efficient delivery mechanisms.
Administrative data on the cost of programmes and take-up are key to understanding what works in supporting incomes. However, current focus is on marginal improvements in, for example, the take-up of the winter fuel allowance. Given the payment is made once a year, near-time information is unlikely to be any more helpful than the routine collection and analysis of administrative data.
It remains to be seen what other lessons are learned from the COVID-19 crisis. However, the increased use and development of near-time data will undoubtedly be beneficial.