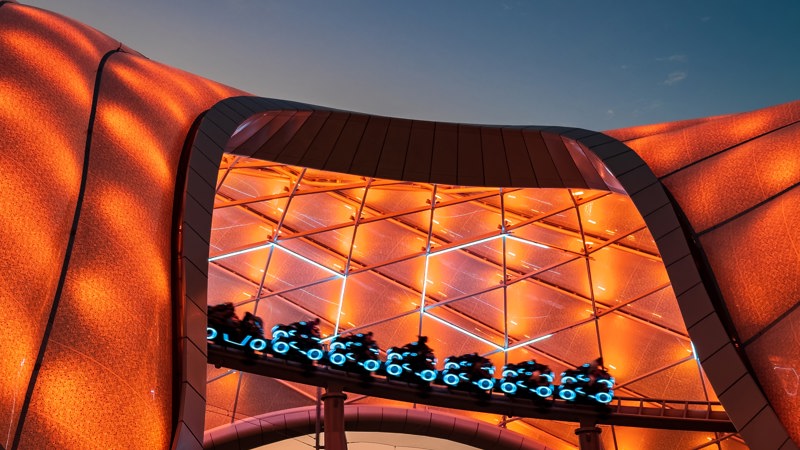
Every day the world’s use of digital data consumes vast amounts of energy, from data stored and processed on cloud servers and in on-premises data centres, to data used on industrial and consumer devices and machines.
Moving, processing, copying and storing data all require copious amounts of energy as well as other natural resources such as water, minerals and land. It is not just our personal or professional use of data that matters - it is also the system-wide ripples that are generated when we do things like ping a photo to friends or train machine learning (AI) models in our place of work.
The International Energy Agency estimates that every bit of internet protocol traffic associated with consumer or business use leads to another five bits of energy being consumed in the actual data centre hosting the workload.
Hyperscale data centres that power the world’s IT clouds have made major strides in energy efficiency over the last decade, through impressive improvements in computing power, virtualisation technologies and storage. But there is scant cause for complacency. Data volumes are projected to leap from about 79 zettabytes in 2021 to 181 zettabytes by 2025, driven by massive growth in next-generation technologies such as AI, machine learning, the internet of things, virtual and augmented reality, computer-generated holography and, at some stage, quantum computing. At the same time, incremental improvements in energy efficiency through the use of public cloud technologies are likely to become harder to achieve.
Figure 1 – Volume of data created, captured, copied and consumed worldwide from 2010 to 2024 (zettabytes)
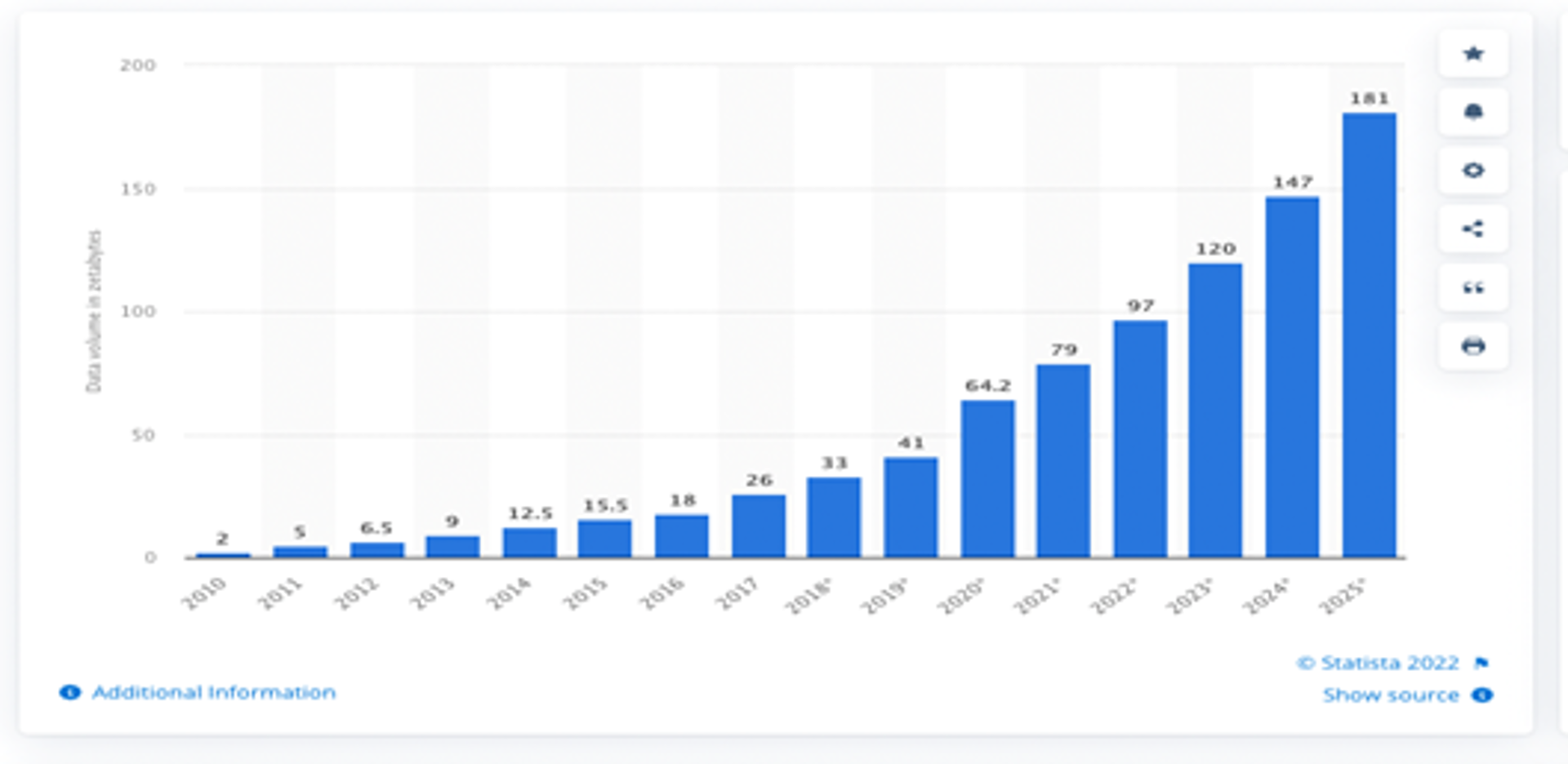
Besides its environmental footprint, data are widely seen as critical to bringing about improvements in governance, decision-making and technological innovation that could help combat climate change and meet other environmental challenges in the future. Despite this, data policy remains a neglected component of sustainability strategies.
One issue that is ripe for exploration in this context is data waste: digital data that is no longer needed due to age, degradation of quality or redundancy in some form. The extent of data waste is hard to measure precisely, but a variety of sources suggest it is a significant problem for businesses. Either data is unnecessarily duplicated or valuable information is being stored but cannot be retrieved and used productively.
On the other hand, there are often very good reasons why organisations store more data than they need on a day-to-day basis — for disaster backup, regulatory compliance purposes or as training datasets for AI tools, for example. What’s more, organisations may want to keep some types of data in the expectation that they will find new uses for them in the future. As geo-spatial, social media, sensory, biometric and other forms of data evolve over the next decade, it is not hard to imagine innovative and exciting use cases with strong societal benefits. Examples include new ways to track biodiversity loss and changes in land use, as well as better ways to identify the health impact of climate change and pollution.
The challenge, therefore, is what to discard and what to retain. It seems natural to ask what role innovative economic tools and incentives could play in optimising data usage to reflect its societal benefits and costs. We look at three things that could form part of an emergent economics toolkit for considering data waste.
Emerging methods for pricing data
For in-house data, one possibility could be to adopt shadow pricing of digital data sources, with internal charging mechanisms to reflect the costs, including energy, of storing large volumes of data in databases and on servers. An alternative approach, borrowed from financial markets, could be the use of options contracts, with a third party taking out the option to buy the data at a specified future date. Such contracts would also have the virtue of revealing the market-determined future option value of that data.
Options pricing admittedly has limitations. It depends on the seller having ownership rights to the data. It may work less well for public or semi-public datasets where ownership rights are more opaque and societal benefits more diffuse. More problematically, markets have short time horizons and may struggle to anticipate long-term future use cases. A case in point is the UK Coal Authority, which has started re-examining 100-year-old colliery maps to help energy companies identify geo-thermal sources of energy that could provide sustainable heating for towns in the north of England. Would market mechanisms at the time have foreseen such use cases? It seems unlikely.
In these instances, alternative decision-making frameworks could be developed that value data according to factors such as its uniqueness, the likelihood it could be easily replicated in the future and the extent to which its use is limited to specific purposes. The public sector might also want to pay attention to private, public and third-sector data where future societal benefits could accrue to those other than the data owner.
Assessing the value of shared data
Data sharing can be doubly beneficial for sustainability, by reducing the need for costly replication of data (depending on how it is shared) and by enabling shared solutions to societal and business problems. Yet perhaps the majority of socially useful data is not shared, either for commercial reasons or because organisations lack the resources to extract and disseminate it.
Economics can contribute through improved and more frequently updated and better frameworks for the valuation of shared data — at the macro and sectoral level. One of our recent studies for the Open Data Institute found that a 25% increase in data sharing could boost GDP for the world’s 20 largest economies by somewhere in the region of $47bn to $118bn. Another of our studies assessed the economic benefits of integrated data sharing for the UK’s Climate Resilience Demonstrator (CReDo), which brings together water, energy and telecoms network providers to pool climate data and modelling. We estimated that over the period 2020-2050 CReDo could generate economic benefits in the range of £6m to £13m across East Anglia and £81m to £186m (in constant prices).
Creating optimal choice architectures for data use
The third element of a sustainable data policy could be the greater use of nudge mechanisms and incentives to promote the socially efficient use of data. Optimal choice architecture has, for better or worse, become increasingly prevalent in online markets, with AI-based recommendation engines shaping the products, services and choices we are presented with. While still controversial, online choice architecture and digital nudges have proved to be effective in certain aspects of digital safety and online content moderation. Examples include harnessing the power of the crowd to enforce norms and standards in online gaming, or employing conversational bots on social media that ask users to pause before submitting aggressive or inflammatory comments. Given that the environmental impact of most data choices is largely invisible to individuals, better online choice architecture could play an important role in changing people’s behaviour, for instance by highlighting the environmental cost of downloading a movie versus streaming it, or by reminding users to delete multiple previous copies of a file as they write a report.
Optimal choice architecture can also play a part in regulatory and policy design. For example, it could incorporate financial or regulatory incentives for data waste reduction or for the sharing of data by enterprises, especially in socially beneficial areas such as scientific research and the environment.
Data policy remains a neglected but important component in the fight against climate change. Systematic use of innovative economic principles and tools applied to data — around pricing, incentives, sharing mechanisms and valuation techniques — could promote more sustainable data usage, bolster socially useful innovation and help enterprises and governments advance more rapidly to net-zero goals.