Viewed from Mars, it might seem bizarre that policy-makers in western economies should be panicking about low productivity, when AI is rapidly fulfilling forecasts of the automation of anything between 10 and 30 per cent of existing jobs (and alarming even the technology geeks).
What’s the explanation of this “productivity paradox”, and are we humans (yes, this isn’t written by a bot) worrying about the right things?
If economists are divided about whether economic growth is still a good thing, there’s no equivocation about productivity. You have to be an extreme Good Lifer to believe that achieving more for the same amount of work, or the same amount for less work, isn’t a positive achievement. In the words of the economist Paul Krugman, “productivity isn’t everything, but in the long run it is almost everything.”
So the poor productivity performance of western economies (dropping to miserable in the case of the UK) has long been a cause for concern and the subject of multiple government and academic studies, commissions and investigations. But are we obsessing about the rear view? Is AI about to change the productivity picture forever? And are the main challenges it presents economic - or social?
Let’s start with the productivity puzzle. Most analysis is focused on labour rather than capital productivity, not least because as a rule of thumb, real incomes can’t rise if output per person doesn’t. But comparisons of output per person don’t tell you much about productivity unless you adjust for the proportion of the population that isn’t working.
And even then, perfectionists can argue that output per person employed is a less precision-targeted measure than output per hour worked, because the working day (or year) varies from country to country. So that’s the measure used by the Organisation of Economic Co-operation and Development in its Compendium of Productivity Indicators 2023 (see chart below).
But this adjustment isn’t foolproof, because of the different ways in which countries actually record working hours. And OECD is even less certain about how best to measure “output” economy-wide for the productivity calculation. It offers a choice, between gni (gross national income) and gdp (gross domestic product) per hour. That’s because both suffer from distortions when it comes to the calculation of productivity; gni includes foreign investment income, which is not “produced” by that country’s current workforce; while gdp - but not gni - includes the profits of the multinational companies headquartered in each country, even if they are repatriated abroad. Such profits loom disproportionately large in the gdp of certain small economies such as Ireland or Luxembourg.
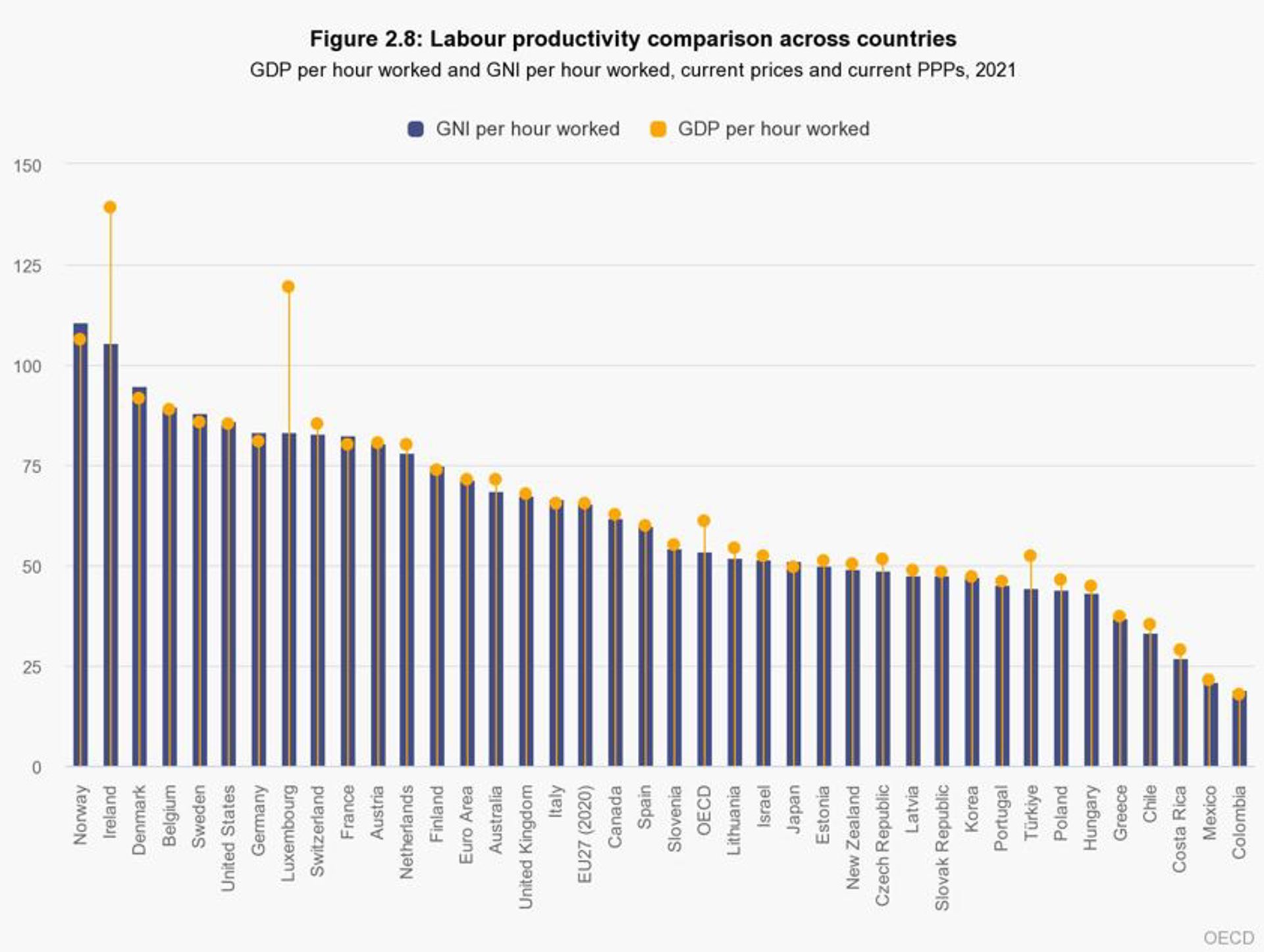
Source: OECD (2023) Compendium of Productivity Indicators 2023 (Accessed on 6 April 2023)
So, the OECD on the whole favours gni.
But for all the quirks in the different indices of productivity, they give a pretty clear and consistent message: most countries’ rate of growth has slowed down markedly. In the UK, for example, through most of the second half of the twentieth century, output per person employed rose at about two per cent a year; since the millennium, it has dropped to below one per cent. Germany has experienced a similarly dramatic decline, as has France - and even the United States, though its labour productivity growth continues to outpace Europe and Japan.
Which takes us to the paradox: why hasn’t the extraordinary “third industrial revolution” boosted productivity, in the way the first and second did?
Well, some simply conclude that for all the hype, the digital revolution just wasn’t as significant as the steam engine, electricity or motor transport. Others argue more convincingly that it certainly was, but that the knock-on effects on employment tended to diffuse the effect on the national figures.
The story goes like this. Automation enabled by IT continued to do what economists said it would: namely, it reduced costs and increased demand, not only for the goods concerned but for other goods and services as well, generating new sources of employment. (Look at any schedule of vacancies, and ask yourself how many of those jobs even existed half a century ago.)
This shift in employment went, at least in part, to some of the sectors least affected by new technologies, notably personal services, and\or where productivity is extremely hard to measure. For example, it appeared to fall dramatically in financial services in the UK following the financial crisis. With a drink or two in your hand, you can have a heated debate about whether massive fees charged by investment bankers reflected massive overcharging or massive productivity, but they are just one example of an industry without a physical product where output, quality and price are hard to disentangle. At the other end of the income scale, if care workers have a phone app that enables them to cut journey times between patients, that’s higher productivity: but if they shorten their visits, is productivity up or quality of output down?
A country’s industrial mix clearly weighs on the productivity figures (and even on our ability to make meaningful calculations of them), but comparisons within industries can tell you more about a country’s performance than comparison between sectors. Not least because different countries’ performance in the same industries quickly show up a key factor: the level of investment, which has been particularly concerning in the UK.
Self-evidently, increasing the capital-labour ratio tends to increase labour productivity. It of course also raises the question: what’s happening to capital productivity? Here the evidence is more mixed, with a general decline followed by flatlining in most major economies. But the critical factor has been what’s happened to “multi-factor productivity”, or the efficiency with which you deploy your particular mix of capital and labour.
This is calculated as a residual in growth accounts, and so is a bit of a catch-all, but it acts as another important clue to the productivity paradox - in almost all countries, not just the UK.
The figures suggest that whatever the opportunities offered by the digital revolution so far, we’ve proved patchy at implementation. There’s plenty of real world evidence to support this view. The corporate landscape is littered with the rusting wrecks of failed IT projects, and public sectors have been painfully slow to adopt and adapt. As well as failing to achieve parallel innovation in organisational structures and management processes, it’s also argued that we’ve been less good at “diffusing” innovation from leaders to laggards than we were in previous industrial revolutions. After a boost from the IT revolution in the late 1990s (more evident in the US than in Europe or Japan), the overall impact on multi-factor productivity slowed down.
So what is it about AI that will change the story? The Reith lecturer and computer scientist Professor Stuart Russell argues that our definition of AI should include all machines and functions capable of autonomous decision-making. The difficulty, as he acknowledges, is that his broad definition of AI encompasses everything from ChatGPT to the thermostat on my radiator. In its brand-new White Paper, the UK Government uses a narrower definition: processes that are not merely “autonomous” but also “adaptive”. (So this wouldn’t include my radiator unless it started taking its own decisions as to how warm I should want to be.)
The key feature of these new forms of AI - such as generative AI or machine learning - are that they are capable of overcoming a lot of the problems that have held back multi-factor productivity. Machine learning can self-solve implementation problems. Rather than require changes in management processes and business models, the new AI tools can simply replace them. So these category-different forms of AI are capable of transforming economic performance, in a way “basic” IT never could.
A recent study by Goldman Sachs estimated that AI would replace 300 million jobs in the next 10 years, and (conservatively) calculated the boost to US labour productivity at 7 per cent. And these include jobs much farther up the management chain, so some argue that this will be a scale of change that will severely test economies’ ability to adapt and generate new jobs.
This looks too pessimistic. We are a long way from a labour surplus, with demand from an ageing population growing for services which are essentially human. As Camilla Cavendish points out in Extra Time, her excellent book on policies for an ageing world, the Japanese have pioneered the use of robots to make old people feel less lonely, but their limitations are pretty obvious. People still need people. And meanwhile those who pointed to labour’s falling share of national income during the first decades of the digital revolution have been somewhat confounded by a reversal in the past five years.
Economically and socially, therefore, freeing up people to fulfil other demands still looks like a very Good Thing. Hence the UK Government’s desire for the British economy to become an “AI superpower”, seeking to attract clusters of innovators. But trying to do so by offering regulation that is “lighter touch” than in (say) the European Union brings its own challenges.
In its White Paper, the UK Government rejects the specificity of the pioneering EU approach to AI regulation in favour of one to be based on “principles”, to be applied by existing regulators. The focus on “outcomes” makes good sense, since prohibiting specific types of algorithms is likely to be a wild-goose chase after fast-flying innovators. And a sense that the General Data Protection Regulations, hatched in the EU but still applied in the UK, drove too much grit into the business machine (as well as hampering the third sector) may explain the Government’s reluctance to dial up regulation of AI. But trying to use a loose federation of existing sectoral regulators to mitigate all types of risk from AI on the basis of general “principles” that blur economic and social risks may prove challenging.
With the overarching guidance of the Competition and Markets Authority, these sector regulators may prove up to the job of managing the risks to competition. Not all the risks to civil society, however, can be assessed with the tools of economic regulation, and are dangerously likely to fall between the cracks.
Most people’s conscious experience of AI is still limited to unintelligent chatbots on the “customer service” bit of a retail website. That feels irritating rather than threatening (and barely amounts to a competitive advantage). But ChatGPT and the like introduce a new sophistication. And we are already less consciously experiencing some less innocent applications of AI: for example, its use to screen job applications, or the crossing of a fine line between “personalised” offerings and manipulation. The UK White Paper acknowledges the dangers of bias in generative AI. And we have already seen the development of emotion-tapping algorithms that, in Professor Russell’s warning words, tend to make us more extreme versions of ourselves. It is not clear that a loose network of sectoral regulators is best suited to keep abreast of these dangers.
So: encouraging AI industries to “cluster” in the UK may well help to boost our multi-factor productivity, which would still, unequivocally, be a Good Thing. And tried and tested approaches to economic regulation may prove useful and useable against potential competition issues. But the potential threats to civil society require much more regulatory thought. Some of those technology superstars calling for a pause may have axes to grind; still. their warnings should be taken seriously. The last time and place we talked so blithely about “light touch” regulation was in the financial sector. When was that? Oh, just before the banking crisis.
Guest Author: Baroness Sarah Hogg, Former Frontier Economics Chairman