In recent years, poker has been revolutionised by applying insights from game theory, a standard tool in economics for modelling consumer and firm behaviour. This article tells the story of how and why game theory entered the poker world. It also explains what businesses, regulators and economists themselves can learn from poker’s game theory revolution.
How do competition regulators predict how a merger between two companies will impact the prices paid by consumers? How do tech giants and governments design auctions to allocate online advertising space, telecommunications spectrum and emissions trading permits? In each case, part of the answer lies in modelling firm behaviour using game theory.
Game theory is the study of situations where the best choice (or “strategy”) amongst alternatives depends on the choices made by others. Not all situations are like this – when you choose what to have for breakfast you probably don’t care what other people are having for breakfast – but such interdependent choices are common in real world games (hence the name “game theory”). For example, in football a penalty-taker might choose whether to shoot left, right or straight down the middle of the goal depending on what direction she thinks the goalkeeper will dive. Economic situations often have the same characteristic – for example firms choosing their product prices may wish to undercut their rivals’ prices just slightly.
Game theory analyses these situations using a concept called a Nash equilibrium, named after the Nobel prize-winner John Nash (you may remember the movie A Beautiful Mind, starring Russell Crowe as Nash). A Nash equilibrium is a stable situation where all participants are making the best choices they can, given the choices of their rivals. For example, in the merger models used by competition regulators, a Nash equilibrium means that all firms are choosing the prices that maximise their profits given the prices chosen by other firms. Today, game theory and Nash equilibrium underlie how economists think about interdependent choices and thus underpin much of modern microeconomics.
Applying game theory to poker
Poker seems a natural fit for game theory since the choice of when and how much to bet depends on your own cards but also the cards of your opponents and how they plan to play those cards. Indeed, one of Nash’s predecessors, John von Neumann, tried applying game theory to poker back in 1944.
For the next 60 years, however, game theory was mainly ignored by poker players. They knew about odds and probabilities to some extent, but generally relied on rules of thumb based on practical experience. Strong players were characterised by a good “feel” for poker and a mastery of table talk, body language, psychology and other intangibles. As James Bond says in the 2006 film Casino Royale: “In poker, you never play your hand, you play the man across from you.”
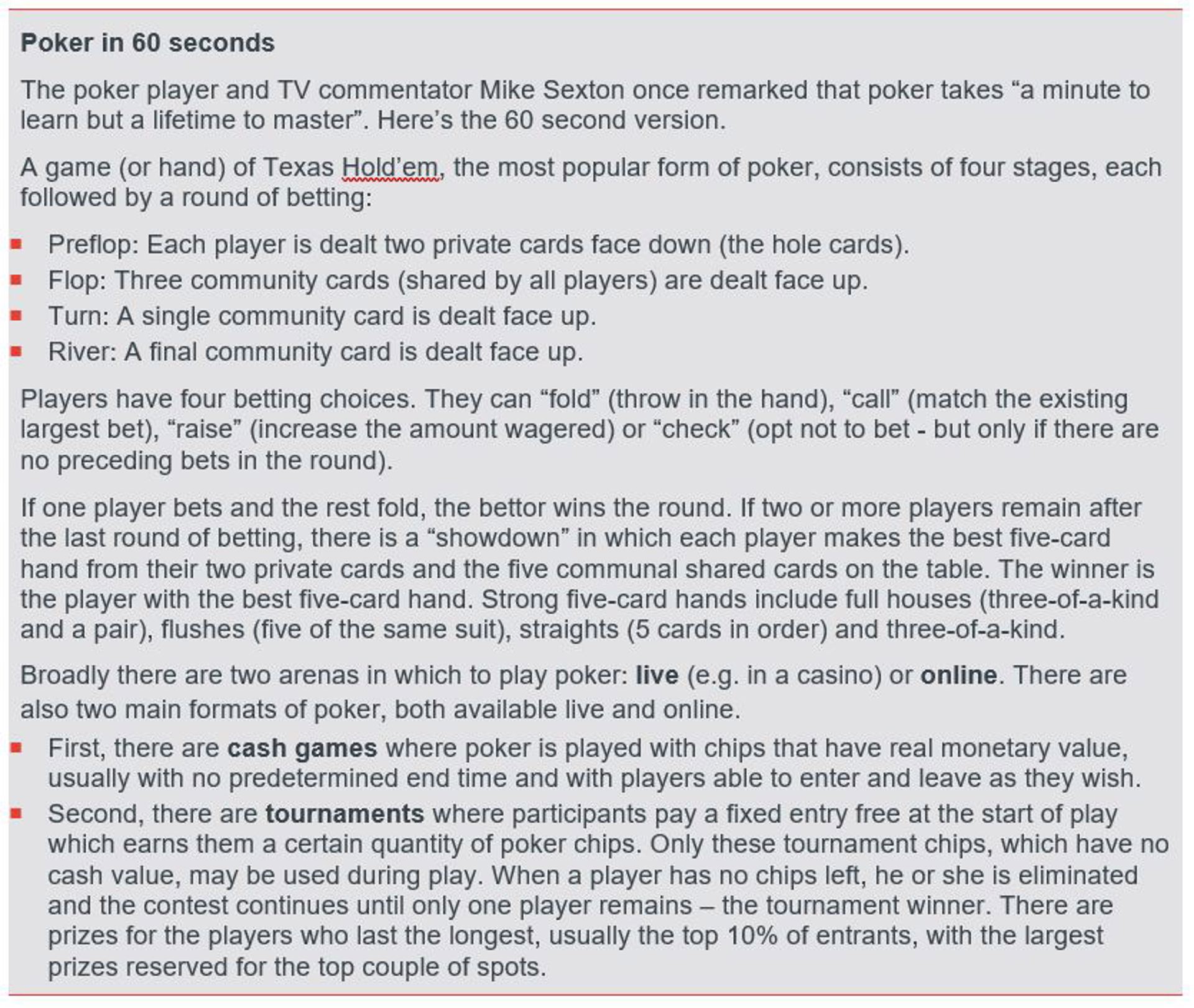
The poker boom
To understand how things changed, it is worth going back to the birth of modern poker in 2003, when the aptly named Chris Moneymaker, an accountant, won the World Series of Poker (WSOP) Main Event in Las Vegas (basically poker’s world championships) and earned a cool $2.5m. Moneymaker's unlikely win captured the imagination of the public (he qualified via a $39 stake in an online poker tournament, rather than paying the standard $10k entry fee) and helped sell poker as a spectator sport.
Following Moneymaker’s victory, TV poker audiences grew rapidly, helped by the introduction of “hole card cameras”, miniature cameras positioned beneath specially designed poker tables with glass panels allowing TV viewers to see the cards dealt face down to each player. In 2007, poker became the third most watched “sport” on TV in the US. The number of WSOP entrants increased from 839 in 2003 to 8,773 in 2006, which is roughly the same as today (8,569 in 2019).
Internet poker, launched in the late 1990s, enjoyed a similar boom. Major online sports betting brands diversified into poker with the most popular sites drawing peak traffic of tens or even hundreds of thousands of visitors. Poker’s sudden popularity (and the money to be won) attracted new players to the game and meant that many of them, if they were good enough, could turn “professional” and make a living from playing full-time poker.
Old School vs New School
The rise of online poker created a divide between old-school players who had made their careers playing live poker in casinos and a new school of online players who learnt the game on the internet.
Compared to the old school’s reliance on “playing the player”, the new online crowd were frequently considered "maths" players who based their decisions strictly upon probabilities and odds. They tended to be young – Annette Obrestad, a Norwegian, began playing online at the age of 15, with the screenname “Annette_15”. But they were also experienced. In 2007 when Ms Obrestad won £1m at a tournament aged 18, she noted, “I’ve played thousands of tournaments online. Most professionals only play 60 or so live tournaments a year so I think I have an advantage there.”
It was the new-school players who took game theory seriously, particularly the idea of Nash equilibrium strategies (which players referred to as “Game Theory Optimal” strategies). As applied to poker, a Nash equilibrium obtains when all players are choosing “strategies” (recipes of how to play in each possible situation – when and how much to bet, when to call, when to fold, etc.) that maximise their monetary winnings given the strategies used by the other players. If you could play a Nash equilibrium strategy in poker, you would never lose money in the long run (once the luck of the cards had evened out), no matter who your opponents were. Even if the other players knew your strategy, they couldn’t beat you – your strategy would be “unexploitable”. And John Nash had proved back in 1950 that such Nash equilibrium strategies must exist in poker.
Of course, it is not enough to know that the perfect strategy exists – you also need to know what it looks like. But even for the most powerful computers, finding a Nash equilibrium strategy in poker has so far been unattainable – with 1,326 combinations of starting hands, 254,251,200 possible combinations of community cards and few restrictions on bet sizes, even two-player poker has around 10160 hypothetical situations.
However, that didn’t mean game theory had nothing to offer poker. At first, new-school players calculated Nash equilibrium strategies in simplified models of poker using little more than pen and paper. Then, as computing power improved, they started using game theory “solvers”. These online calculators, available for a few hundred pounds, weren’t powerful enough to find a Nash equilibrium in a full game of poker, but they did make it much easier to zero in on Nash equilibrium strategies in specific situations or in simple poker models. Players could plug in key inputs (e.g. number of betting rounds to go, stack sizes, community cards, allowed bet sizes, the possible cards each player could hold) and the solver would come up with the appropriate Nash equilibrium strategies. Players could then look for patterns and try to understand the logic behind these strategies – how often should they be betting, what sort of hands should they bet on, etc.
Raising standards
The new generation of game theory-savvy poker players revolutionised the way the game was played. Three changes stand out.
- Aggressive bluffing. Perhaps most noticeable was how often new-school players bluffed (i.e. bet with weak hands). When the likes of Ms Obrestad started bluffing very frequently in the early 2000s, they were thought of as crazy; if they were winning, it must be down to luck. Previously, players had intuitively thought they should bet mainly good hands and bluff only a bit. Game theory showed this approach was wrong, particularly in the early stages of hands. In game theory models, the optimal ratio of “bluff bets” to “value bets” was usually about 2:1 on the flop (when three community cards have been dealt with two more to come). This means that when you bet, you should be twice as likely to have a weak hand as a strong hand. The logic is twofold. First, bluffing means your opponents will need to call your bets often to stop you winning with weak hands; hence you’ll frequently win a lot when you have a strong hand. Second, many hands you bluff with can improve and become a strong hand when more community cards are dealt. That means you can get away with bluffing a lot when there are still more community cards to come.
- Short-stack play. When players have relatively few chips, decisions can often be boiled down to a choice between going all in (i.e. betting all your chips in one go) or folding. When poker can be simplified in this way, game theory provides recommendations on exactly when to go all in and which hands to fold. New-school players memorised these recommendations intensively, giving them a significant advantage over rivals who ignored the maths and instead relied on “feel”.
- Mixed strategies. Game theory often recommends mixed strategies – randomly choosing what to do with a given hand in some situations – to avoid becoming too predictable. This was particularly important for players on the internet, where opponents could use tracking software to analyse their play and look for weaknesses.
According to one new-school player, “As poker has become more popular over the last 15 years or so, players have improved dramatically, to the point where it’s very difficult to consistently beat the game without game theory knowledge in your corner…old-school players made their millions from exploitative play, whereas almost all players making millions now depend on poker game theory.”
While game theory was mainly the preserve of a mathematically inclined minority in the 2000s, today there is strong demand from casual poker players to understand and deploy game theory. As such, a poker training industry focused on game theory has sprung up. Poker books now have titles like “Play Optimal Poker: Practical Game Theory for Every Poker Player”, and there is any number of online training courses and apps that promise to teach aspiring poker players the secrets of game theory.
Perhaps the ultimate end-state for poker will come when computers are able to find Nash equilibrium strategies for the whole game (i.e. “solve” poker). Artificial intelligence (AI) is being harnessed to that end. One AI poker software, named “PokerSnowie” and costing $99, was “trained” by playing against itself billions of times. Another, called “Libratus”, beat four poker professionals in a 120,000-hand Brains vs. AI challenge match over 20 days, using algorithms that were designed to approximate a Nash equilibrium. Although poker is still too complex to be fully “solved” for now, players often assume poker AI is starting to get close to an actual Nash equilibrium of the game.
That is not to say game theory has all the answers for human players. Poker game theory works best against strong opponents, since Nash equilibrium strategies maximise your winnings only when other competitors are “rational”. Against recreational players who make systematic errors (such as not bluffing enough), adopting a Nash-type strategy means that you are not necessarily maximising your winnings. This has sparked a big debate in the poker world on whether you should aim to play a Nash-based or an exploitative strategy (involving systematic deviations that can be exploited by good players, leaving you vulnerable, but that help you win more against weaker players). In reality, there probably isn’t much conflict here: good poker players need both strategies. Game theory provides the baseline from which to adjust, just as traditional economic theory with rational consumers acts a baseline that behavioural economics then adjusts to account for common biases that people exhibit.
Betting on the future
The story of game theory in poker provides a reminder of the benefits of traditional economic models. Even though game theory cannot capture every detail of a poker hand, it can still provide practical insight – just as merger models, for example, do for competition regulators. But poker may also point to the future for economists. While game theory, with its strong assumptions about players being “rational”, does not always do a good job of describing how humans played poker in the past, it probably does a good job of describing high-stakes poker today and an excellent job of explaining how two poker AI programs play against each other. Poker AI players have either been designed to mimic economic theory or, alternatively, seem to have taught themselves to operate in ways that satisfy economic theory. If AI satisfies the idealised assumptions of economic models better than humans, and AI starts taking a larger role in consumer and firm decision-making (e.g. by guiding consumer shopping choices and setting prices for firms), then perhaps economic models of mergers, auctions, collusion, and price discrimination will do a better job of describing AI-dominated economies than human-dominated economies?
Some caution is required here – poker may not be a good example from which to extrapolate and AI may not in fact end up taking a larger role in consumer and firm decision-making. As Frontier’s David Parker has explained, AI is most likely to be employed in a situation where there is a large amount of data. This allows for the development of:
- Good predictive models: by using large amounts of “before” data, AI can develop good “after” predictions.
- Classification analysis: for example, by using lots of pictures of faces, AI can learn to identify a face in a new picture (which is essentially another version of predictive analysis).
- Game playing devices: in games with static, well-specified rules such as Chess and Go, AI can repeatedly play against itself to develop an understanding of optimal strategies.
The use of AI in poker very much sits in the third category. But much of “real life” decision-making is messier and more dynamic than poker. AI predictions will be inaccurate when limited data is available or when the ‘rules of the game’ change (new firms enter a market, customers’ tastes change etc.).
Given the uncertainty about the future role of AI, we’ll have to wait and see whether the game theory revolution in poker is a sign of things to come in the real economy…or if the economists are just bluffing.