
Looking ahead to the next water price review
In December 2019 Ofwat, the industry regulator for water companies in England and Wales, released its final determination for the latest price control round (PR19). In this, it set out for each company the allowed revenue and expected service levels over the next five years. A key part of this process involved assessing the efficiency of water companies, and ultimately their cost allowances, through the application of cost benchmarking. The benchmarking of the residential retail control, one of the 6 price controls Ofwat sets, is of particular interest owing to the complex demographic drivers underlying why some people default on their water bills. In this article, we take a closer look at what the benchmarking of bad debt and debt management costs – a significant part of the residential retail control – might look like in the next price control round, PR24, and the crucial role demographics could play.
What makes benchmarking of bad debt costs different?
As a general principle, regulated water companies are expected to have efficient costs, so that customers aren’t charged for a company’s inefficiency. One of these costs is the failure of households to pay their bills on time, if at all, and the subsequent pursuit of payment in these cases. In effect, these bad debt costs are socialised among all the firm’s customers. So knowing the efficient level of such bad debt and debt management costs is critical.
There is however a tension the regulator faces between being too stringent or too lax in the bad debt cost allowance it sets. If the allowance is set too high, customers face higher bills and water companies may come under less pressure to manage their bad debts more efficiently. By contrast, if the cost allowance is too low, then water companies may not be able to finance their business even if they are well run. Determining the efficient level of bad debt, and incentivising companies to reach that standard, is therefore one of Ofwat’s key functions.
This tension naturally exists for most of the cost allowances Ofwat sets. The interesting aspect of bad debt benchmarking is trying to determine why unpaid bills vary between companies. Here, two sets of factors are at play: first, the particular demographics of customers that explain their propensity to default; and second, the efficiency of water companies in recovering bad debt. What benchmarking does is to try and control for, using econometrics, the first set of characteristics. This is because the incidence of these factors varies between the regions different water companies serve. It then assigns the remaining variation in bad debt to company efficiency. This method, after controlling for differences in the characteristics of the customer base, allows for like-for-like comparisons of water companies and ultimately informs Ofwat’s decision on their cost allowances.
What the above highlights is the importance of taking into account all the complex demographic drivers behind why customers default on their bills, but, crucially, focusing only on those drivers which fall outside each firm’s control. If this approach was not followed, then the resulting variations in bad debt would be a combination of company efficiency and the demographic characteristics which haven’t been controlled for. In effect, this would mean some water providers (and ultimately their customers) would be rewarded through their cost allowances for appearing more efficient than they actually were while others would be penalised for appearing less efficient – giving rise to the tensions discussed above.
How could the current approach be improved?
For PR19, Ofwat used a number of measures to try and explain differences in bad debt and debt management costs between companies. These included average bill size, two different proxies for the average propensity to default and a measure of geographic mobility. These are all calculated as an average for each water company’s geographic area of coverage.
All of these measures have a clear economic rationale (e.g. if you’ve defaulted previously, you’re more likely to default again) and generally perform well in Ofwat’s econometric analysis. However, they likely fail to capture some of the ways in which people are driven into financial hardship. For example, Ofwat uses the income score of the Index of Multiple Deprivation as one of its two proxies for hardship. While this metric is highly relevant, it is probable that the propensity to default is also linked to additional dimensions of deprivation, such as those listed in Figure 1 below, and which are not currently included in the regulator’s cost benchmarking models.
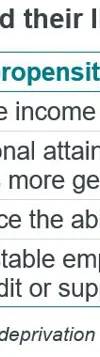
A separate but linked concept is that of vulnerability, or how likely individuals are to suffer detriment due to their personal circumstances. The source of vulnerability could either be longstanding (such as long-term illness) or temporary in nature (such as recent bereavement or short-term illness). Even if some extended measures of deprivation were included in the benchmarking models, they might still not pick up on the demographic factors relating to vulnerability, which are also likely to impact the propensity to default.
A more general point is whether the current benchmarking approach of taking company-level averages is best suited for an event that affects a relatively small number of people and which has common drivers across the country. Given that the variation in demographic factors across company averages is likely to be much lower than the variation within company areas, the current method effectively ignores this additional within-company variation, ultimately making it harder to estimate robust models. Further, this approach yields only a few observations, and the measures that Ofwat does use generally do not change over time and are not regularly reported. This raises the question of whether a more geographically detailed, less aggregated method using customer demographics could generate more accurate and fairer bad debt cost estimates, potentially solving some of these issues.
What might PR24 look like?
A new cross-company approach to customer demographics could take into account some of the extra dimensions of deprivation and vulnerability discussed above while also recognising that averages can be arrived at through very different underlying distributions. Developing new proxies for these measures is likely to be challenging but, with the Index of Multiple Deprivation as a template, it is certainly possible.
Taking this further, the creation of a specific Index of Water Deprivation which incorporates the specific demographic characteristics of those customers who default on their water bills could be a natural next step. This could be founded on a consistent and comparable annual survey of households in each water company area, which is then combined with the wider information that firms already hold on their customers. Benchmarking could then either be done at the household level for each company (with the added benefit of increasing the total number of observations) or aggregated up to the water-company level. This would hopefully allow more detailed demographic information to be obtained, resulting in a more precise assessment of the true driving forces behind bad debt costs and ultimately water company efficiency.
In short, a deeper understanding of the demographic causes of why people default on their water bills could serve to advance this area of regulation further. We believe PR24 is a great opportunity to scope out new, fairer ways of assessing bad debt costs using customer demographics while allowing companies to better understand their customers and target support to those most in need.