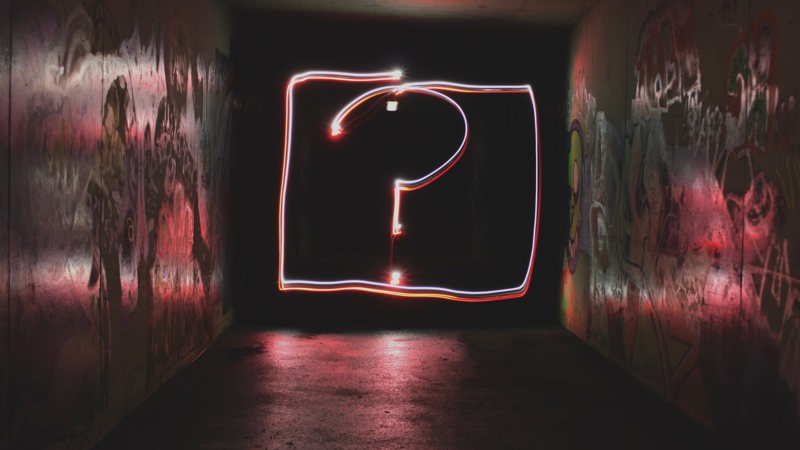
How economics can improve the regulation of online harms
A live streaming of government troops opening fire on protesters. A video blog questioning scientific evidence around the causes of the covid-19 pandemic. Personal comments about the body appearance of a fashion influencer on Instagram. A heated debate on an online forum that suddenly turns personal.
Every minute of every day, social media organisations need to make a dizzying array of decisions about the content posted on their platforms, often at high speed and with little context, to prevent or mitigate a variety of online harms. While there is no precise definition of the term ‘online harms’, it generally encompasses everything from child abuse, terrorist propaganda, graphic violence, hate speech and extreme pornography to online bullying and harassment. Some types of online harms are per se illegal, whereas others are legal but adjudged harmful depending on the context. Some commentators also extend the definition to include elements of fake news and disinformation.
The explosion in user content
Such harms also exist in the offline world, of course, but the digital revolution has vastly altered their speed, scope and nature. An old-fashioned physical bulletin board usually had a human as gatekeeper or moderator. In real life people don’t usually insult each other face to face, no matter how entrenched their different views. Media plurality laws, professional journalism and standards of editorial integrity are significant constraints on disinformation in the published media. The key change wrought by the digital revolution is the explosion in user-generated content, much of it behind the guise of perceived anonymity. Digital media allows anyone to act as a political commentator, a scientific expert, an arbiter of fashion or, at the more extreme end, a spreader of hate speech or propagandist for violent causes. Moreover, the volumes of user-generated content are staggering. Consider that more than 500 hours of content are uploaded to YouTube every minute, 1,050 Instagram photos are uploaded every second and 6,000 tweets are sent every second, amounting to an average of 500m tweets in a single day.
Keeping up with the context
While social media companies invest millions in both human and AI-enabled content moderation, they face several major challenges. One is the changing nature of online communication and the growth of internet culture: opinions are often communicated by memes, GIFs, slang words or videos rather than by text. Context is also critical but often difficult to evaluate: transmission of a violent incident by a news organisation or a civil rights group, for example, could well be justified in the circumstances if it highlights a wider issue or injustice in society. Even seemingly innocuous symbols can take on more sinister meanings. One example is the cartoon-strip character Pepe the Frog, which over time has evolved from a somewhat apathetic fictional housemate to a meme associated with alt-right groups and racist memes and imagery.
Is it you? The rise of deepfakes
Moreover, new technologies are continually outflanking the ability of content moderators to respond to online harms. A particular area of concern relates to a type of deep-learning algorithm, technically known as Generative Adversarial Networks, that can produce often highly realistic fake videos of individuals, both living and deceased. While deepfake technology has legitimate commercial uses in film and content production, it has also been used for online harassment and the spread of disinformation. In one case a deepfake video of Barack Obama shows him making disparaging remarks about Donald Trump; in another case a deepfake Instagram of Mark Zuckerberg has him claiming that his true goal is to manipulate users. According to research by deepfake detection company Darktrace Labs, the number of deepfakes in circulation doubled from 7,964 to 14,678 between December 2018 and December 2019, with the vast majority pornographic in nature and focused on female celebrities. Even more alarmingly, generative adversarial networks can be used to alter the underlying data (or hashes) of harmful content to make it appear innocuous to algorithmic content-moderation systems - in one case tricking an algorithm into identifying an abusive image as that of a typewriter instead.
Bringing the economics toolkit
Given the multi-faceted nature of online harms, approaches to content moderation and regulation call for input from a variety of disciplines: not only technologists but lawyers, ethicists, linguists (to understand context, translation and meaning), psychologists and political scientists (to identify harms to democratic accountability, election integrity and media plurality). To date, however, the role of economics in the online harms debate has been relatively neglected. In this article we argue that economics has much to offer both in understanding the impact of online harms and in devising effective solutions and regulatory methods. In particular, we highlight three areas where the economist’s toolkit can be put to good use, viz:
- Identifying the different risks and costs of content-moderation decisions;
- Evaluating the potential costs and incidence of different regulatory proposals to address online harms, so that better targeting can be achieved;
- Analysing the role of incentive structures to identify optimal content moderation structures.
1. Evaluating the risks and trade-offs of content moderation
Algorithms are increasingly being called upon to act as gatekeeper in content moderation and regulation of various online harms - including hate speech, fake news, sexual abuse and terroristic propaganda. A report by Ofcom highlights several ways in which algorithms can improve content moderation. These include flagging potentially harmful content before it is even posted (by comparing it against a database of reference images and keywords); by evaluating the content history of users on a site; by assisting human moderators in prioritising harmful content for review; and by lessening the potential trauma for human moderators through partial blurring of disturbing content.
Yet there are still significant limitations to algorithmic content moderation, leading to different risks and associated costs. One concern is false negatives - failing to spot a potentially harmful post. The flipside is the potential for false positives - labelling a post as harmful when it is actually innocuous or justified in the context. A high rate of false positives has prompted concerns about ‘overblocking’, the accusation that algorithmic content moderators may err on the side of caution by taking down difficult-to-assess content that is actually harmless or justified.
Here economics can help illumine the choices and trade-offs involved. Figure 1 below shows the ‘indifference curve’ or trade-off curve for a social media platform using algorithmic content moderator to detect abusive deepfake images. The rate of false negatives is shown on the y-axis, while the rate of false positives is shown on the x-axis. The curve is downward sloping because, for a given state of technology, reducing the rates of false negatives can only be achieved by accepting higher false positives. The curve is relatively flat because the social media platform has a strong preference to avoid false negatives - in other words, to avoid letting a deepfake image slip through the net, with the regulatory fines, private legal action and extensive reputational damage that entails.
Figure 1: Illustrative trade-off for abusive deepfakes

Figure 2 shows a situation where the trade-off is more evenly balanced. In this case the false negatives are terroristic propaganda - Al Qaeda or Daesh, for example - that slip through the net, causing embarrassment to the social media platform and drawing the public ire of politicians. However, the costs of false positives are not inconsequential - for example, the blocking of content of a humanitarian organisation highlighting the human impact of the war in Yemen. In this case the platform could experience reputational damage and accusations of stifling public debate.
Figure 2: Illustrative trade-off for terrorist propaganda/graphic images

Many other variations are possible. The actual decisions taken will depend not just on the preferences of the platform operator, but also on the overall budget for content moderation and the relative costs of different inputs such as human and AI systems (not shown).
Moreover, these trade-offs could shift, depending on a variety of factors, as shown in Figure 3. The advent of better detection technology, for example, could reduce the rates of false negatives and positives simultaneously, or at least lessen the trade-off. By the same token, new deepfake technologies - for example, video-enabled holograms, or detection-avoiding algorithms - could shift the trade-off curve outwards. Other relevant factors could include the size and scope of the social media network, influencing the speed of transmission of content. The virality of the network - the extent to which speed and clickability of content are important to the platform’s business model - can also shift the curve either way. In general, platforms which rely on highly viral content to generate clicks and advertising revenues may have to accept higher rates of both false positives and negatives, even if this is somewhat detrimental to their reputation.
Figure 3: Better detection technology or better avoidance technology shifts trade-off

2. Evaluating economic impacts of regulatory proposals
In light of the mounting concerns about online harms, governments and regulators across the world have been stepping up with a variety of new laws, codes of practice and regulatory systems. Given the disparate and often poorly understood nature of online harms, however, there is a real danger of regulatory missteps or ineffective targeting of proposals. Economics can play a crucial role here in analysing the impact and incidence of such proposals, leading to recommendations on how to promote better targeting and more effective outcomes.
A case in point is the UK Government’s Online Safety Bill, which puts forward a range of legislative proposals to address a wide variety of online harms. Among other things, the Bill is set to introduce a new duty of care on social media platforms, to be overseen by an independent regulator with power to issue fines, take enforcement action, request transparency reports and approve industry codes of conduct.
For online harms regulation to achieve its objectives while minimising the risk of unnecessary economic burden on businesses and third sector organisations, there are at least two key issues to be considered:
- Which activities and organisations are in scope of the regulation; and
- The risk that the regulation may divert company resources away from the most cost- effective ways of dealing with potential harms.
First, regulation needs to apply widely so that harmful behaviour does not simply move from regulated to unregulated online services. However, this needs to be balanced against the fact that the risk and scale of harm vary substantially across services, and that the resources available to companies to prevent and remove harms also vary greatly.
Second, given the very large amount of online content, achieving cost-effective ways to screen this content to prevent and identify harms is crucial. Automation can help but it is not a one-size-fits-all solution and it may not always be cost-effective, particularly for smaller companies. Our analysis suggests that annual cost of creating and maintaining an automated system to detect a relatively well-defined category of harm (e.g., extremist content) is around £7m. This is likely beyond the capacity of many small, fast-growing platforms. For example, our analysis of Beauhurst data identified 812 fast-growing companies that could be in scope of regulation. The total amount of equity investment raised by these companies ranged between £700,000 and £1.2m. Careful calibration of the effective incidence and impact of new content-moderation can contribute to better policy design while mitigating adverse impacts on fast-growing sectors of the economy.
3. Nudges and nods: understanding the role of incentives and optimal design
A third area where economics can improve the regulation of online harms is through behavioural economics and a better understanding of how incentives influence online behaviour. One promising area of application is nudge theory, developed by Richard Thaler and Cass Sunstein in their 2008 book ‘Nudge: Improving decisions about health, wealth and happiness’. A highly influential concept in policy circles, the basic idea behind nudge theory is that small and relatively inexpensive changes in choice architecture - the way in which options are designed or presented - can have big impacts on human behaviour in the aggregate. The classic example would be supermarkets displaying fruit or low-calorie snack bars rather than chocolates near the check-out aisles, reducing impulsive buying and making it easier for shoppers to default to the healthier option.
Given the expense of online content moderation, nudges can be a low-cost way of reducing the frequency of lesser online harms, such as abusive language in online forums. Online bots can ask users to reconsider the offending part of their post, suggest more acceptable revisions or introduce a slight time delay to give the user a cooling-off period before posting. Some forms of AI-based content moderation draw on the power of the ‘crowd’ or ‘user community’, for example online bots that encourage users to flag unacceptable language or behaviour.
Such a system has been used by Riot Games to moderate player behaviour in their League of Legends online game. The AI team first assembled data on 100m votes of the user community on cases of reported bad behaviour to assess relative severity. Armed with these rankings, the makers then trained an AI system that penalises players for bad behaviour - such as harassing or discriminatory language - and rewards players for good behaviour. The initial analysis revealed that the vast majority of cases flagged were typically one-offs, with a relatively small proportion of cases coming from persistent offenders. It is reported that due to the introduction of the system, incidents of sexism, racism and homophobia fell to 2% of all games, and verbal abuse was reduced by 40%. The reinforcement effect seemed strong, with 91.6% of users who were initially flagged not repeating the offence.
Experimental and game-theoretic approaches can also shed light on optimal design for online content moderation. Fiala and Husovec (2018) report the results of an experiment designed to reduce the problem of overblocking, or excessive compliance, by content moderators in cases of reported harmful content or possible copyright violations. As noted above, such overblocking can occur because incentives are heavily stacked in favour of deletion for online platforms, which can face large fines or legal suits for failing to act quickly to remove contested content. By contrast, content posters are often unable to assert their rights or are poorly informed about rights of appeal. This asymmetry in incentives means that non-harmful content is often inadvertently blocked. Fiala and Husovec show experimentally that a relatively small design change, introducing an independent alternative dispute resolution (ADR) mechanism to which posters can appeal for a refundable fee, reduced the rate of incorrect takedowns by platforms in the first instance from 39% to 19% and then, on appeal, to 10%.
Conclusion
Despite the social, political and legal nature of the online harms discussion, economic concepts and tools have much to contribute to the debate. In particular, economics can identify the shape and nature of the trade-offs that must be faced in content moderation, help evaluate the impact of different regulatory proposals and assist in the design of optimal content-moderation systems and incentives. In fact, it is likely that we have only scratched the surface in terms of the application of economic concepts: other possible applications could include better techniques for valuing the negative externalities of online harms; deploying measures of market concentration and dispersion to identify political fragmentation and polarisation from fake news; or analysing market failures stemming from the dilution of informational quality in online markets.
With online harms growing day by day, there is no better time to start putting the economics toolkit to good use.
This article was co-authored with Mark Purdy, Associate.