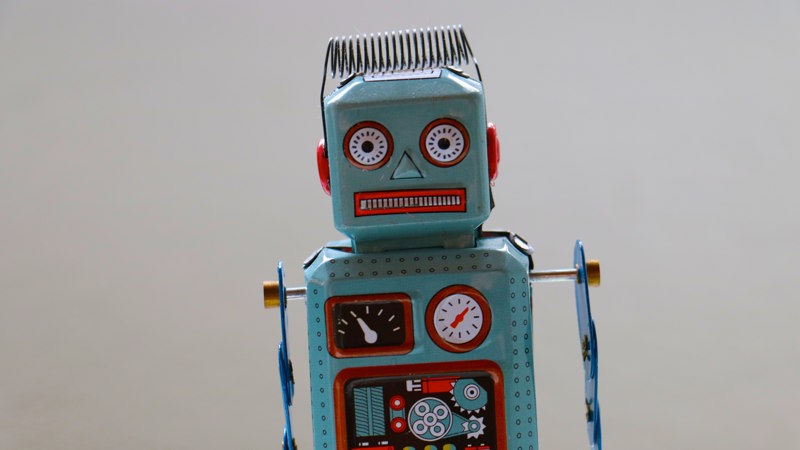
Behavioural analytics in regugation
Sundar Pichai, Google’s CEO, made the case in his speech at the World Economic Forum in Davos this year that “artificial intelligence is probably the most important thing humanity has ever worked on. I think of it as something more profound than electricity or fire."
This is a bold claim, but it does reflect the potential seen in AI algorithms, machine learning, big data and data science by business and government. It is not surprising, then, that regulators are also looking at how they can harness these techniques to carry out their work more effectively.
A couple of definitions might be helpful to start with. Artificial intelligence (AI) is a wide field of study that has the radical goal of replicating human decision-making capabilities. Machine learning (ML) is just one facet of AI. It refers to the use of algorithms to analyse information, often from datasets based on millions of observations, to spot patterns and make predictions. It is used in many different types of processes, such as lane recognition in car safety systems. But regulators are increasingly interested in deploying these techniques to analyse customer and firm behaviour.
“Alexa, what is it you machines can learn?”
The techniques themselves have been around for a long time. Insurance underwriting, credit risk modelling and direct mail targeting have relied on data and predictive algorithms since computers made them possible. Recently, though, the big tech companies have given ML a much higher profile as behavioural analytics becomes part of our daily lives – from the recommendations we see on Netflix to how we interact with voice services from the likes of Google and Amazon.
Regulators, working in different sectors with businesses that hold vast quantities of accessible, customer-level data, are naturally starting to look at the potential for ML to help them better understand how consumers behave. But how exactly?
We can broadly break down ML techniques into two categories of behavioural analysis:
Spotting patterns. Also known as unsupervised ML, this involves searching for patterns or clusters in data. Algorithms look for distinct groups of customers – minimising differences within groups and maximising differences between groups. Tesco was a pioneer in using these techniques in the retail sector thanks to its Clubcard, launched in 1995, which gave the retailer huge amounts of data on what each customer bought over time. Using this analysis, it could identify missing products that certain customers might be interested in trying, or were likely to be buying elsewhere. And it could improve decisions of what products to stock in large and small stores by better understanding different shopping ‘missions’.
Making predictions. Predicting behaviour based on data is at the heart of many ML applications, including modelling credit risk and recommendations on everything from catch-up TV to groceries. This is also known as supervised ML, and there are many algorithms that are used to ‘train’ a model to enhance its predictive power. These can improve on traditional prediction techniques, such as statistical analysis, as they are able to identify more complex relations. For supervised ML to be effective, however, large amounts of data are required to test and improve the predictive capability of the algorithm.
As well as projecting the likelihood of a particular event for a consumer or company, ML techniques are increasingly able to identify the key factors determining the prediction, potentially yielding valuable further insights.
Putting the machines to work
ML can have a place in the regulatory toolkit to complement existing economic and analytical methods. Whilst the techniques are powerful and have many commercial uses, the challenge is to make the results meaningful for regulatory applications. ML can help in two main ways.
- First, by strengthening the understanding of consumers and the companies that regulators oversee, ML can improve the diagnosis of some regulatory issues. It can also filter and select issues for further investigation.
- Second, in combination with insights from behavioural economics (BE), ML can be used to develop, test and refine regulatory interventions so they are better targeted and more effective. In that way, the costs and unintended consequences of the interventions are reduced.
There are many potential applications, as shown in box below.
Potential application | Sector example |
Avoiding the average. Often regulatory investigations look at averages across markets and consumers. It may be that the issue at hand is a concern only for some groups, but this may be hidden in averages or because customer/consumer segments have been over-simplified. Pattern-spotting techniques can quickly identify specific types of behaviour and segments. Any potential regulatory concern can then be considered separately for each group. |
Financial services: Understanding the characteristics and behaviour of consumers who regularly use unarranged overdrafts can sharpen the diagnosis of any consumer impact. Telecommunications: Improved understanding of the characteristics and behaviour of those consumers who remain with their mobile communications providers after the end of the period required for the cost of a subsidised handset to be recovered. |
Targeting. One of the main commercial uses of predictive analysis is to target communication at specific groups where it is likely to be most effective. Regulators have tended not to use such targeting when they intervene in markets. Instead, they have preferred to apply consumer-wide approaches or to use simple groupings (e.g. older customers) to guide their interventions. ML could be used to develop scorecards that identify customers who are most likely to change their behaviour or benefit most from a nudge, reducing the cost and increasing the effectiveness of regulatory initiatives. |
Energy: Regulators have sought to address concerns about customers who do not switch from higher-priced standard tariffs to cheaper fixed-rate tariffs by introducing prompts and communications. ML could be used to develop predictive models to identify customers most likely to benefit from different types of prompt. Telecommunications: The UK regulator, Ofcom, publishes on its website a report explaining the quality of service of different providers of broadband services. ML techniques could be used to better direct such information to consumers most likely to be affected by poor broadband quality.. |
Detecting unusual behaviour: Pattern-spotting can also be used to identify unusual behaviour that may indicate specific risks such as fraud or miss-selling that occur in a small number of outlying cases. By looking at large volumes of data, ML can help recognise outliers without the need for a prior theory bout the nature of the consumer segment or behaviour. |
Water: Metered customers are at risk of paying higher bills if they have leaks or use water inefficiently. ML can be used to identify customers with unusually high water usage who should be helped to reduce their bills. Financial services: The UK’s Financial Conduct Authority regulates 56,000 firms. By monitoring a range of public and private data, firms exhibiting unusual behaviour (e.g. financial metrics or social media mentions) can be selected for further investigation. |
No regulatory robots, please
In most regulatory contexts, the relevant questions are about consumer behaviour (e.g. how do different consumers use a product and why) and economics (e.g. how would companies be expected to set prices in a particular market). These questions cannot be answered just by crunching data, so there is no chance of ML or AI techniques replacing regulatory decision-making and judgment anytime soon.
Many decisions are needed to construct models that can be used effectively, and these are tasks for human experts. They include carefully specifying the regulatory question. Often an issue to be investigated could be clear-cut (e.g. customers who may be paying too much). In such cases, simpler techniques can be used to test the hypothesis and the extent of any problem. Identifying which customers are being charged over the odds can be straightforward, in which case deploying ML to spot clusters of high payers would be unlikely to be helpful. It is usually also important for regulators to understand causality, and not just correlation between variables. A model that finds that certain types of consumers who receive text alerts are less likely to use an unarranged overdraft does not mean that sending text alerts to other consumers will reduce unarranged overdraft usage. It may just show that consumers who are more careful with their money sign up for text alerts. Other behavioural research, such as using randomised control trials, may be needed to establish causality.
And where predictions are made it will be important to be able to explain the economic intuition and context underlying them, particularly in situations where the outputs of models may be challenged. An expert witness who justified her view on how firms would set prices based on unprocessed ML output alone would not make a compelling case. This evidence would need to be complemented with analysis and other approaches, such as more traditional statistical techniques, that aim to identify causal relationships.
Behavioural Economics and Machine Learning
It will take time for machine-learning techniques to become a ready part of the regulatory toolkit, judging by the experience with behavioural economics over the past ten years. After a surge in academic and then popular interest, there were some early adopters of BE in the regulatory community who wanted to develop a better understanding of customers. This was followed by steady progress as regulators learned how to harness BE techniques. Some, such as the Financial Conduct Authority and the Competition and Markets Authority, are developing their own capabilities in ML. Regulators in other sectors are likely to follow.