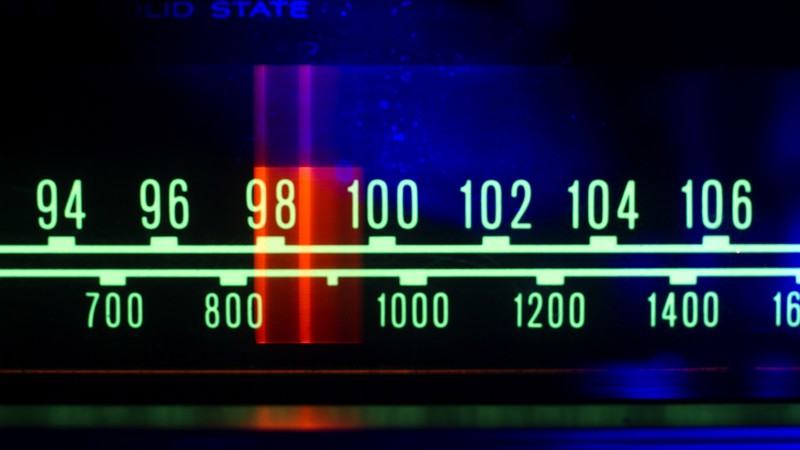
A new paper by our Competition and Data Science economists explores how machine learning can be used to estimate damages in cartel cases - a complex and high-stakes task in competition law.
Published in the Journal of European Competition Law & Practice (registration required), the piece examines how machine learning can complement traditional econometric methods by increasing predictive accuracy and reducing modelling time. It also flags important limitations, such as the ‘black box’ nature of some algorithms and challenges in demonstrating causality - both key concerns for courts and authorities.
Written by Will Carpenter, Anna Lane, Joshua Hia, Iain Boa and Steffen Reinhold from Frontier, with Martin Spindler from the University of Hamburg, the team set out a framework to assess when machine learning could be helpful, and how to apply it rigorously in legal settings.
Our Competition economists are increasingly advising on cases where economic and digital expertise must go hand in hand. From assessing algorithmic collusion to supporting tech regulation and private enforcement, our work brings together robust economics and a deep understanding of AI’s real-world impact.
The new paper is part of our ongoing research into the role of advanced analytics in antitrust enforcement.
Click here to read the full paper on the Journal’s website now (registration required)