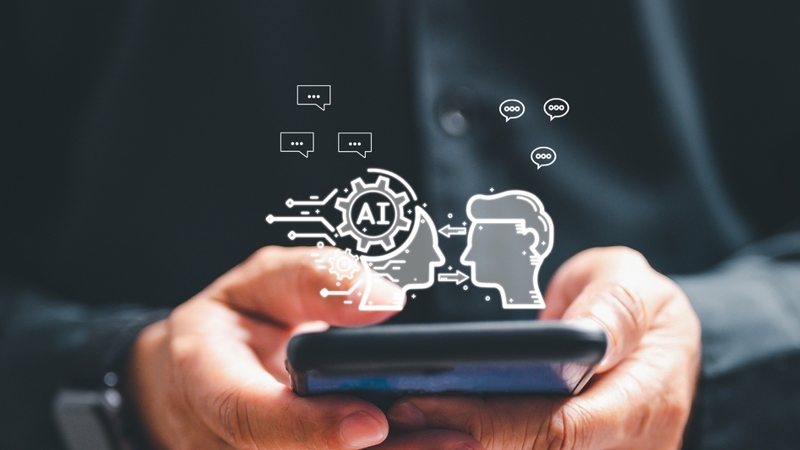
At Frontier Economics we continue to closely examine the evolution of Generative AI (Gen AI) and have watched the latest release of ChatGPT with particular interest.
ChatGPT-4o brings with it a significantly improved voice-based interface. OpenAI has also fully integrated ChatGPT’s data analysis functionality, allowing users to upload data and prompt the app to explore and analyse the data without the need for analysis skills. If the demos are to be believed, working with tools like ChatGPT will soon be akin to having a new expert colleague in the room.
The death of the data scientist?
Gen AI is already transforming how we approach data science and economic analysis at Frontier. For example, it can produce new code and debug problems amazingly well. More recently, our Data Science team has been testing the new data analysis features and learning valuable lessons. Our testing shows that ChatGPT excels in generating analyses and charts. It also writes, on the face of it, very eloquent and logical commentary on its analysis. However, as we highlighted in a recent viral LinkedIn post, ChatGPT is not poised to replace data scientists just yet. A closer review of the commentary shows that ChatGPT cannot correctly interpret and understand results.
Our experience continues to be reflected in the wider academic research on Gen AI. In the last few weeks, Stanford University’s Reglab has published new research that evaluates three of the most popular bespoke AI tools for legal research. It found that the tools do reduce errors compared to general-purpose AI models like GPT-4. But even these bespoke legal AI tools still provide incorrect answers an alarming amount of the time, with rates ranging from 17% to more than 34%.
Understanding human biases in the interaction with technology
These examples show that a significant risk of hallucination (producing incorrect or nonsensical information) remains even in the most advanced AI tools. As businesses push to roll out AI, there is a clear risk that a user may miss these errors either because they are underinformed or under pressure, especially when the apps interact like a human colleague. It also poses significant questions for businesses that are building or deploying Gen AI applications, especially if dealing with consumers.
Thinking about how businesses can mitigate these risks goes right to the core of how humans engage with IT applications
Academic research into human-IT interaction shows how behavioural biases influence human engagement with technology. Behavioural biases are human traits that can have positive and negative consequences. They can arise from mental shortcuts (heuristics) that help us process information quickly, both consciously and unconsciously. However, they can lead to errors in reasoning due to subjective perception, shaping how individuals interpret reality and affecting rational decision-making.
These traits apply to how humans engage with Gen AI and can be used by developers to increase user engagement, but they also entail risks if not managed carefully:
- Authority Bias: People tend to trust information presented by authoritative sources, including computer applications, without questioning its accuracy or validity. Gen AI often produces responses as eloquently written, unambiguous summaries or essays, typically without citing sources. Despite this, authority bias means users may not question the information provided.
- Anchoring Bias: This occurs when an individual’s decision making is affected by the initial information provided (the "anchor"). You might want to ask yourself how often you have questioned the first route given to you by Google maps? In Gen AI, users may anchor on the first answer given, even if that information is irrelevant or misleading, and this incorrect information may be reinforced through subsequent exchanges or chats with the app.
- Confirmation Bias: People tend to seek out information that confirms their existing beliefs or opinions, or at least to consider information that aligns with their beliefs to be a closer reflection of the truth. When Gen AI provides information that chimes with their preconceived notions, users are more likely to accept it without critical evaluation. The user's biased prompts can lead the AI to generate responses that reinforce their prior beliefs, creating a vicious cycle and potentially generating an incorrect overall result or information for the individual.
- Automation Bias: Humans often rely heavily on automated systems, assuming they are infallible. However, when the user experiences a bad outcome, this can quickly turn into rejection and aversion because they hold the automated system to higher standards than a human operator.
- Overconfidence Bias: People often overestimate their ability to critically assess information, leading them to accept Gen AI outputs without thorough evaluation of their accuracy or reliability.
All of these biases apply to human interactions with IT systems. There is another human trait which is particularly relevant: anthropomorphism, the human tendency to ascribe human traits to non-human entities. There is evidence that humans have a higher level of trust in technology that has human-like attributes and tend to be more accepting of information presented with anthropomorphic tendencies. This can be seen in the rapid and widespread adoption of virtual personal assistants like Alexa and Siri. This emotional connection and familiarity can increase acceptance of Gen AI outputs.
Human behavioural traits will be at play whenever people engage with GenAI apps, especially with an ever-improving human-like interface. As we move into a world where humans will be able to hold verbal conversations with Gen AI apps, the risk that they unquestioningly accept the responses is only going to get bigger.
Mitigating risks in Gen AI deployment
To maximise the transformative benefits of AI, businesses must identify and mitigate this risk whether they are deploying apps for use by internal teams or external customers. If AI applications lead to mistakes and bad outcomes, users will rapidly lose trust and confidence. This impact, and the responsibility for it, is underscored by the UK Competition and Markets Authority’s (CMA) recent update report on Foundation Models and AI:
“If consumers lack trust and confidence in AI and AI-driven services, they are less likely to use them and benefit from what they offer, and the innovative and disruptive benefits of organisations or individuals using AI may not reach their full potential. We have already seen concerning arguments made by businesses that they are not responsible for the outputs of AI-powered tools used on their websites, and similar developments in future could damage consumer trust in AI-related markets.”
At Frontier, we have been learning practically how to mitigate these risks by developing Gen AI applications ourselves, such as our recently launched Assisted Merger Intelligence tool. Through our work we have identified three things that are critical to minimising the impact of misinformation from Gen AI apps:
Reduce the risk of hallucination: App developers can use several techniques to reduce the risk of hallucinations and inaccurate answers from Gen AI models. These include fine-tuning foundation models, which involves further training the AI with data specific to the business context. Another method is Retrieval Augmented Generation (RAG), whereby the AI generates responses using only a relevant subset of data.
Maximise data quality: Techniques such as fine-tuning and RAG work better if they are based on good-quality data. If Gen AI models are retrained on messy data with errors then these will be perpetuated in their responses. The principle of “garbage in – garbage out” applies to Gen AI apps. The performance of Gen AI models can also be improved through limiting their scope of focus, i.e. it is much harder to build a Gen AI app that accurately answers questions about legal precedent across all countries and sectors compared to one area of law in the UK.
Address behavioural bias in user interactions: Businesses deploying Gen AI must thoroughly consider and test how people will interact with their app, taking into account how they behave in real-world settings. By understanding the behavioural science, developers can create more intuitive and reliable user experiences. This knowledge should inform every aspect of the app, from UX design to training and communications, and be tested on a continual basis as both the technology and their users’ understanding evolve. This approach ensures that users can effectively and safely engage with the app, enhancing both usability and trust and maintaining compliance with AI and consumer protection regulations.
Conclusion: A holistic approach to AI
The evolution of Gen AI holds immense potential to transform business operations. However, realising this potential requires a careful, informed approach that considers the inherent risks. Businesses must engage with AI responsibly, understanding and mitigating the biases and errors that can arise from human-AI interactions.
At Frontier, we are committed to guiding our clients through this transformative period. By drawing on our extensive expertise across behavioural economics, data science and AI regulation and policy, we can help our clients harness the power of Gen AI while navigating the ethical, societal and regulatory considerations that accompany it. If you want to learn more about how we can assist your business in deploying Gen AI applications responsibly, please get in touch.