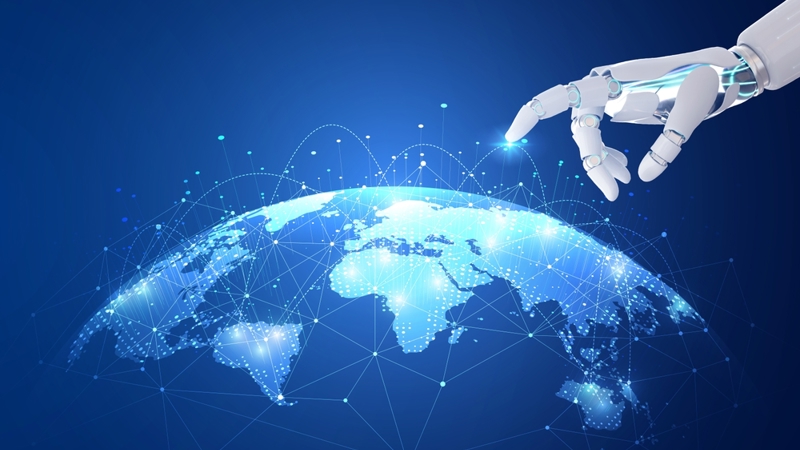
Can AI improve telecom operators’ profitability?
Pressure to boost profitability to more sustainable levels
The profitability of many telecom operators is currently relatively low, especially in Europe. They have been exploring a number of ways to restore earnings to more sustainable levels, for example through divesting tower assets. At the same time, the telecoms sector is experiencing significant technological challenges with the roll-out of more advanced networks and fundamental changes in how networks operate. One of the important shifts that is taking place is the rapid emergence of Artificial Intelligence (AI), which is expected to improve how telecom networks are run and maintained. And telecom networks are themselves important enablers of the use of AI, including generative AI.
A lot of the discussion to date around the impact of AI has focused on how it may improve the sector’s efficiency, e.g. via network automation, predicting faults and the use of chatbots in customer support (see Figure 1). However, there is also a question as to whether AI may create new revenue opportunities for telecom operators. Of particular note, SK Telecom appears to have gone all in on AI with it aiming to generate £14bn in revenue from AI by 2028, as part of its strategy to become a global AI company.
Figure 1 Key benefits of AI in the telecoms sector
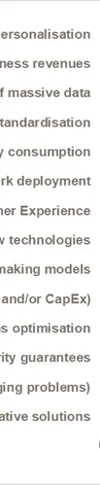
Source: BEREC Report on the impact of Artificial Intelligence (AI) solutions in the telecommunications sector on regulation, 8 June 2023
Note: When assessing whether to deploy a new AI solution, which benefits listed below do you pursue or prioritise? Average rating of survey respondents
Using AI to make telecom networks more efficient
Telecom operators have been rolling out gigabit-capable fixed networks and 5G. The Body of European Regulators for Electronic Communications (BEREC) expects that the application of AI to operational procedures will become the norm in the next six to ten years.
There are at least three main ways in which AI can lead to efficiency gains and improved performance for telecom operators:
- Optimising network performance by deciding how best to allocate network resources, which is partly being facilitated by the virtualisation of networks;
- Avoiding faults and networks outages; and
- Helping to predict where network upgrades are most needed.
All of these factors could ultimately help to cut costs. Improved network performance and enhanced capabilities may also boost revenues by opening up new use cases and by increasing customer satisfaction. For example, AI will facilitate the use of network slicing.
Potential revenue opportunities
In principle, there are at least four ways in which the development of AI could provide telecom operators with increased revenue opportunities:
- The use of telecoms networks could increase owing to mass-market AI consumer applications;
- There could be an increase in demand for enhanced network quality for specific AI business use cases,
- AI could enable more personalised offerings to end-users, improving telecom operators’ sales and marketing; and
- They could develop foundation models and/or generative AI applications themselves.
Increased use of telecom networks from mass-market AI consumer applications
Tech advisory firm Omdia forecasts that by 2025 most network application traffic will involve AI content generation, curation and/or processing. By 2030 it predicts that nearly two-thirds of network traffic will involve AI, with the rapid growth of video and image content.
But will increased data traffic due to mass-market consumer applications result in higher revenues for telco operators? Rising demand is nothing new for telco networks: the advent of smartphones and the development of apps produced a surge in mobile traffic (Figure 3), but as noted this was associated with stagnating ARPUs and low profitability for European telecommunications operators.
Figure 3 Annual mobile data traffic worldwide

Source: Ericsson Mobility Visualizer
Growing demand for enhanced network quality for specific AI business use cases
Telecom operators may have more opportunities in future to increase revenues from specific business use cases. Network slicing, for example, allows operators to offer end-users a more differentiated service. This could particularly appeal to firms that would be prepared to pay more for superior network quality (perhaps facilitated in some cases by edge computing) to meet their specific needs - for example, AI use cases that require high bandwidth, low latency, high reliability and/or high security.
Improved sales and marketing
Telecom operators can use traditional AI to better personalise their marketing, offerings and pricing. In effect, they can use AI to try to predict consumer preferences (based on their past behaviour, among other things) and tailor their sales tactics and marketing materials accordingly to suit individual end-users. To the extent that telecoms operators start offering more differentiated quality of service to different users, AI may be able to help predict the level of network quality that different users would be interested in.
This raises the question of whether some elements of net neutrality rules may need to be re-visited to enable telecom operators to have a better chance of monetising the ability to offer more differentiated and targeted services. According to the European Commission, some large internet access service providers consider that “the current rules and approach to net neutrality do not provide sufficient certainty to enable them to launch services based on network slicing or define specialised services.” Potential providers of foundation models and generative AI applications
Generative AI applications can be either provided as a standalone offering, such as ChatGPT, or integrated into existing services, such as search, social media, operating systems, mobile ecosystems and productivity software. Telecom operators do not appear to be in a particularly strong position to develop such services (or foundation models (the ‘engine rooms’ of generative AI) as they have less expertise in AI/machine learning than Big Tech firms and they are not leading providers of cloud services. Whilst they are arguably strongly placed on access to data, the value of this depends on whether the data is encrypted and whether they have the legal rights to use such data.
On balance, it seems that telecom operators would need a clear change in strategy if they are going to become important providers of foundation models and/or generative AI applications. SK Telecom will provide a good test case of whether this is possible.
The role of partnerships
One option would be for telecom operators to enter partnerships with firms that are more specialised in AI e.g. SoftBank in Japan has a partnership with Perplexity AI to offer an AI tool to SoftBank’s customers, which provides instant answers to questions with sources and citations. Under such an approach, how much value telecom operators can retain from offering AI applications may come down to their bargaining power relative to their potential partners. Telecom operators’ bargaining power with potential partners will likely depend on how many viable partners there are, which will be driven by how the market structure of the AI sector evolves over time.
Policy implications
Collecting, processing and transmitting data is essential to AI services, which helps explain why telecom networks are an important enabler of such services. Their role may become more important as the nature of AI applications evolves, given that:
- Data may increasingly be collected from the edges of networks. To date, most of the data that has been used to train AI models has either come from propriety datasets or has been scraped from the web. That being the case, the data has typically come from centralised sources rather than being gathered from the edges of telecom networks. However, this may change over time given the proliferation of the Internet of Things (IoT), which incorporates a broad range of sensors in more remote locations. This may therefore increase the need for high-speed telecom networks to cover as much of the country as possible (if the data is in a rich format, such as video), including out-of-the-way places.
- Data may increasingly be processed at the edges of networks. Hitherto the processing of data for AI models has also tended to occur in more centralised locations, such as large data centres. Again, this may change somewhat over time, given that a number of applications will rely on low latency and real-time processing. As a result, edge computing may play an important role in facilitating the use of some AI applications, such as improving vehicle safety and analysing healthcare data.
The rise of AI is likely to place increased demands on telecom networks, especially if the gathering and processing of data shifts towards the edges of networks. Existing fibre networks may be relatively future proof given the capabilities of FTTH technology to deal with the bandwidth required by AI applications. However, in the context of weak profitability, mobile operators may have limited incentives to roll out 5G networks, including edge computing, on a sufficiently widespread basis to maximise the use of AI. As a result, there may be a need to refine policy to further incentivise 5G deployment. For example, as 5G technology will support significantly greater differentiation in services that may be needed to support some AI applications, there is a risk that network innovation could be chilled if telecommunication operators are unclear about which services are permissible. Therefore, net neutrality rules and guidance may need to be updated to reflect a world where different types of customers and services offered require different levels of quality of service.